.png)
Almost every organisation has committed recently to making their teams and workplaces more diverse, equitable and inclusive. And more than ever before, there’s a recognition that we need to address inequities at the systemic level: at the level of how we design the interactions between people in communities, public services and organisations.
This reframing and sense of urgency is to be celebrated because for too long we’ve let good intentions give way to quiet inaction.
But that still leaves many organisations a little lost on where to start and what impacts they can expect to have from the changes they might make.
Hiring can be part of the solution, since if you don’t start by attracting and selecting people from more diverse communities, it’s pretty hard to correct for it later on (in fact, statistically, the hurdles become exponentially more challenging, which is why thinking about these issues as organisations scale is so critical).
But what is the impact of shifting your hiring process to one that’s more inclusive? How much can it really improve representation and how quickly?
To help answer this question, we recently did a piece of analysis on how our own data stacks up on improvements to ethnic diversity in one of the most topical and fastest growing sectors: the STEM(M) sector.
With thanks to the New Scientist for featuring these findings in their recent deep diver into institutional racism in the sciences.
Diversity in the STEM(M) workforce
Traditionally encompassing organisations in science, technology, engineering, mathematics (and now medicine); STEMM organisations and the roles within them are central to almost any projection of the future skills workforce.
The WEF’s 2018 Future of Jobs Report found that the bulk of the trending skills lie in STEMM - as shown below.
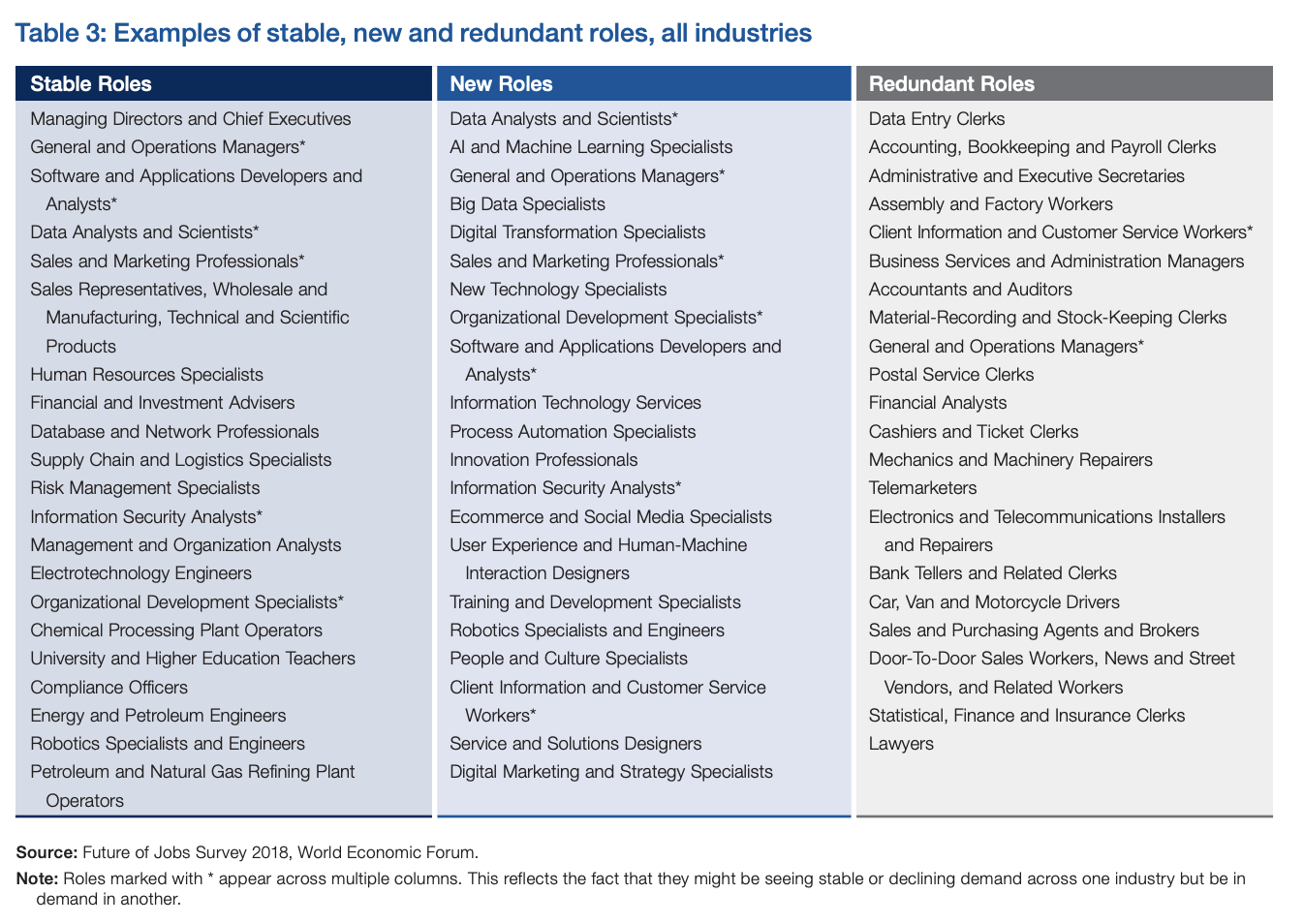

Understandably, there has long been a focus on how to increase the proportion of women and non-binary people in STEMM roles since this data has long been abysmal and in some cases regressing.
But people have also started to ask themselves how STEMM-related organisations are doing at attracting and retaining all types of talent.
Why diversity in STEMM matters
You don’t need to look far to find out why.
The absence of racial and ethnic minority representation in tech and medicine can have catastrophic consequences. For example, we’re now increasingly aware of the impact that a lack of diversity in drug testing has on the (in)effectiveness of many household drugs, not to mention the daily frustrations that people of colour and other underserved groups face with technology that wasn’t built with or for their communities. For a salient example of just one of these, take the failure of this soap dispenser to work equally across two skin tones - this case becomes more apt in the current COVID19 crisis.
So as organisations try to marry the imperative to act with the gap in their current tools they’re likely to be thinking about hiring remedies, including blind hiring.
If you are - you might want to know how much impact debiasing your hiring process can have.
While it’s important to measure multiple forms of diversity (we measure 7 dimensions for example), in light of recent events, we decided to do a deep dive into racial and ethnic diversity in STEMM, and the extent to which a redesigned, debiased and more predictive method of hiring can improve the changes of under-represented groups being hired.
The short summary is: a lot.
Our methodology
First we classified all our UK-based partners into sectors, and pulled out three categories of organisations that touch on the STEMM workforce:
- Core STEMM organisations - these are our clients which are principally in one of the 5 key domains - science, tech, engineering, mathematics or medicine
- Financial sector organisations - these are financial sector clients (including VCs, private equity and others), of interest because a high proportion of their roles are in quite technical positions which face some of the same challenges as core STEMM
- Research and technical consultancies - these are our clients in the think tank, social and economic research and consulting sectors if their primary work involves technical expertise, and who again, have some related features to STEMM organisations
This resulted in about 15 organisations per category, and we then looked at their data over the past year, which represented just shy of 40,000 candidate applications, and several hundred jobs.
The reason we focused on the UK and organisations (as opposed to all our clients worldwide and jobs themselves) is that we wanted to be able to compare success rates to clear benchmarks where the bulk of our clients are. Benchmarks are typically made at a country level and relate to organisation-level classifications.
On the latter point, it’s worth noting that this does mean we’ve included some non-STEMM roles in STEMM organisations (i.e. a communications advisor in a tech company) but also misses some STEMM roles in non-STEMM organisations (i.e. a developer working for the government).
What we found
We’d already known from prior studies that we’d done that 60% of the candidates who get a job through Applied would otherwise have been overlooked in traditional CV/resume hiring processes. We also knew that these ‘needles in the haystack’ are more likely to come from under-represented groups (including ethnically under-represented groups).
Another piece of analysis we did showed that 45% of all of the roles we have helped to fill into UK tech jobs have gone to women or non-binary candidates (compared to an industry benchmark of 30%).
So we shouldn’t have been surprised to find that a debiased hiring platform would show sizable improvements in representation (since, you know, that’s the point of it!). But what we couldn’t have anticipated is just what the scale of the difference was.
As shown in Figure 1 below, if you compare our hire data over the past year to relevant benchmarks, in all but two cases (mixed ethnicity in core STEMM organisations and other ethnicity in research and consulting), the proportions of hires were more diverse through Applied.
Figure 1: Proportion of hires from an ethnic minority background into STEMM-related organisations through Applied relative to benchmarks

It’s notoriously difficult to get a handle on good benchmark data, but we’ve compared our data to three data sources which provide a useful level of granularity needed for meaningful comparisons: the first is the 2018 UK employed population which the Cabinet Office together with the Office for National Statistics compiles for its Ethnicity Facts and Figures project, the second is a measure of the scientific workforce (broken down both with and without the health sector) which was compiled in 2013 for research conducted for the Royal Society.
Those benchmarks show that between 9 and 13% of the total workforce are from a BAME (or Black, Asian or Minority Ethnic) background. But this overall grouping lacks meaning since as many point out, this is a term many people from these communities don’t identify with, in part because it implicitly assumes the experiences of different groups are the same. That’s why unpacking the data to individual groups is so important.
And on that, you see that the Black workforce represents between 2 and 3% of the total workforce, for the Asian workforce it’s 5-7%, for those of mixed heritage it’s around 1% and for those who self-identify as ‘other’ it’s around 2%.
By comparison, through Applied, Black candidates were hired 3-4% of the time, Asian candidates 15-17%, Mixed candidates 0-12%, and Other candidates 1-8%, meaning that overall, between 26% and 38% of all hires came from a minority ethnic population.
As shown in Figure 2 below, this translates into some sizable uplifts. If you take the average of all three groups of organisations and compare to those benchmarks, you see that Applied is helping teams to hire 2.4-3.4 times as many candidates from under-represented ethnic groups.
Figure 2: Comparison of uplift in candidates hired through Applied from an ethnic minority background relative to benchmarks

What next?
This data gives us and our partners substantial reason to celebrate, since the Applied platform is designed simply to try to level the playing field for all candidates by removing the risk that unconscious or implicit bias influences the hiring decision.
And of course this data can’t currently be separated from other factors which could have contributed to an improvement in hiring rates within our partner organisations, but since our platform is their principal tool for making these decisions, there’s reason to believe that at least some of the impact lies in the debiasing of the process itself.
But we also know we have more we can do. For example, as shown in Figure 2, the uplift is especially high for people who identify as having a Mixed heritage, but not as high for Black candidates. Why this is the case requires yet deeper research, including by continuing to delve deeper into how specific application questions that candidates answer could be further improved and working even more closely with our partners on implementing our interview module which helps to remove bias from those stages of the process.
While it’s beyond the current scope of Applied at this point, there is also a lot of important work teams can do to onboard, support, develop and remunerate all talent - including those from minority ethnic backgrounds - more fairly, so that the demographic diversity and potential is realised.
Like all good research, this work poses as many questions as it answers. And together with our partners, we’re committed to that task since, frankly, anything less wouldn’t be enough.