
There’s been a significant rise in the development and use of AI in hiring decisions in recent years...
By 2020, 55% of hiring managers in the U.S. were using algorithmic software and AI tools in their recruitment process.
And according to a February 2022 survey from the Society for Human Resource Management, 79% of employers now use AI for recruitment and hiring.
But beware: AI can have serious negative consequences when it comes to hiring decisions.
Hiring takes time and a lot of manual work.
And so on the surface, AI seems like a godsend for hirers. It can automate laborious manual tasks, quickly identify the best talent and is even relatively affordable.
To an extent, this is true. Once you've invested the time to actually built your algorithm, it will save you time.
However, this efficiency gain comes at a cost...
Using AI to make hiring decisions entrenches rather than reduces inequalities.
How does AI recruitment software work?
Most artificial intelligence tools are designed to predict future outcomes...
This could be a bank using AI to identify patterns associated with fraud, an e-commerce business predicting which products you're most likely to buy or a hiring tool that identifies candidates most likely to succeed in a given job.
To be able to make these kinds of predictions, AI has to be trained using existing data. It can then identify patterns and use this to make recommendations.
This training data is usually split into two sets: one dataset is used to train the algorithm, and the other is reserved for testing it.
AI developers will dictate what goal they're looking to achieve (e.g. finding the most suitable candidates) and then use the algorithm to identify patterns that will help achieve this goal.
In the case of hiring, if you give the AI a database of hired candidates - it will pick out the characteristics that those who were hired tend to possess.
The second chunk of data is then used to see how accurately the algorithm can predict outcomes. Now you have a baseline for what a hired candidate might look like, for example, you can test the algorithm to see if it picks out the same set of candidates who were hired in the rest of the dataset.
AI recruitment tools come in many forms, but all follow the same rough principles. Whether they help with talent sourcing, job posting, data parsing or candidate shortlisting, they all follow the same process of using existing data to predict who the best candidates are likely to be.
Examples of AI recruitment technology:
- Resume scanners
- Sourcing tools
- Game-like online tests
- Video interviewing software
How can AI recruitment software be problematic?
The use of AI in the recruitment process has been making headlines in recent years for all the wrong reasons.
The most high-profile example of this was back in 2018, when Amazon reportedly ditched their AI and machine learning-based recruitment program after it was found that the algorithm was biased against women.
You might be asking how discrimination like this occurs... isn't AI meant to be more fair and objective than human judgment?
Well, the issues lie in the process used to train AI.
You want your AI to spot candidates like those you've already hired? First you'll have to feed it with existing data so that it knows what to look for. The problem is, this existing data is a product of human decision-making.
The way in which it's collected, who (and what details of theirs) are included and the purpose it was collected for (it'll often be past data not collected specifically to train AI) can all have a significant impact on the end outcomes an AI will produce.
If, for example, your data doesn't contain a diverse enough group of candidates, your algorithm is going to reflect this lack of diversity in its predictions.
The fewer data points you have on people from underrepresented backgrounds, the more skewed your algorithm is going to be.
This is why the majority of face recognition algorithms exhibit demographic differentials. What's a differential? It means that an algorithm’s ability to match two images of the same person varies from one demographic group to another.
How your training data has been tagged and classified can also result in biased outcomes.
To create an AI that will predict which candidates will be top performers, you'll need to know what a top performer looks like. What characteristics make them good candidates?
To find this out, you'd look at your existing performance data - this might be past performance reviews/appraisals or data around promotions.
The AI will then try to identify what these top performers have in common to identify matches.
But given the ways in which we evaluate employee performance and promotions are prone to biases (both rapport-building with senior managers and large client accounts tend to skew towards male and white employees) any AI you build off the back of this historic data is likely to perpetuate these biases.
AI is extremely efficient at replicating the sort of outcomes we see in the world...
However, when we consider that a lot of what we see in the world is systemically biased, AI can only entrench these biases, rather than solve them.
Biased AI doesn't just affect hiring - here are some of the problems of its use across wider society:
- Racism embedded in US healthcare - an algorithm designed to predict which patients would likely need extra medical care heavily favoured white patients over black patients
- COMPAS (Correctional Offender Management Profiling for Alternative Sanctions) - which predicted twice as many false positives for black re-offenders than for their white counterparts
- PredPol - an AI intended to predict where crimes will occur repeatedly sent police officers to neighbourhoods that contained a large number of racial minorities regardless of how much crime happened in the area.
- Google Photos Algorithm - this algorithm was found to be racist when it labelled the photos of a black software developer and his friend as gorillas.
What should you use instead of AI recruitment software?
Here at Applied, our mission is to make the recruiting process as ethical and predictive as possible...
Which is why we never use AI to make hiring decisions.
Instead, the Applied Platform uses a collaborative process to improve inclusion and science-backed, proven assessments to identify genuine talent in the fairest way possible.
Want a more predictive screening process? Use 'work samples'
Resume scanning software relies on keywords to spot what it believes to be qualified candidates.
However, when you take into consideration the wealth of research that shows women tend to downplay their skills on resumes, while men will be more likely to exaggerate and include the right keywords - its clear to see how using AI in this context can mirror the inequalities we already see in the world.
The dangers of AI extend beyond just racial and gender inequalities. The U.S. Department of Justice released guidance warning hirers that AI screening tools could be a violation of the Americans with Disabilities Act.
Things like personality tests, AI-scored video interviews, and gamified assessments, fail to consider individuals who may need special accommodations.
Instead of relying on AI to make decisions, the Applied process uses predictive assessment methods whilst removing the biases that usually cloud human decision-making.
Unlike an algorithm, we don't make assumptions about candidates based on their backgrounds or use keywords to determine who gets shortlisted.
All Applied candidates are screened completely anonymously - no names, no work history, no academic credentials and no CVs.
This is because the most fair, reliable way to predict job performance is to simply assess skills directly.
What do we mean when we talk about 'predictive' assessments? Well, predictive validity is a means of testing the accuracy of a given test/assessment used in science and psychology.
Whilst studies on productivity can take years to complete, we can look at the Schmdit-Hunter meta-analysis as a rough guide to what works when it comes to finding potential employees.

As you can see from the chart above: 'work samples' are the most predictive form of assessment for forecasting job performance.
This is what the Applied Platform uses to screen candidates instead of a standard CV-based process.
Work samples simulate small parts of a job by asking candidates to either perform or explain their approach to them.
Below is a real-life example used to hire members of the Applied Sales Team:
Question: You have received a lead from one of the SDR (Sales Development Reps) at Applied. They have arranged an initial introduction with a 50-person B Corp in the food space that will last about 30 minutes.
How would structure the call and follow-up? What would success look like?
By asking candidates both job and organisation-specific questions, we can see how they would think and work through the everyday challenge the role entails... what could be more predictive than asking candidates to essentially perform small chunks of the job itself?
Here are some of the extra steps you'll want to take to make your assessments more accurate and unbiased...
Anonymisation
All humans are prone to biases - it doesn't matter how pure your intentions may be.
Whilst there may be a few 'bad apples who are explicitly biased or prejudiced, the sort of biases that affect things like candidate screens are largely unconscious.
Just a quick glance at a CV can trigger a number of biases...
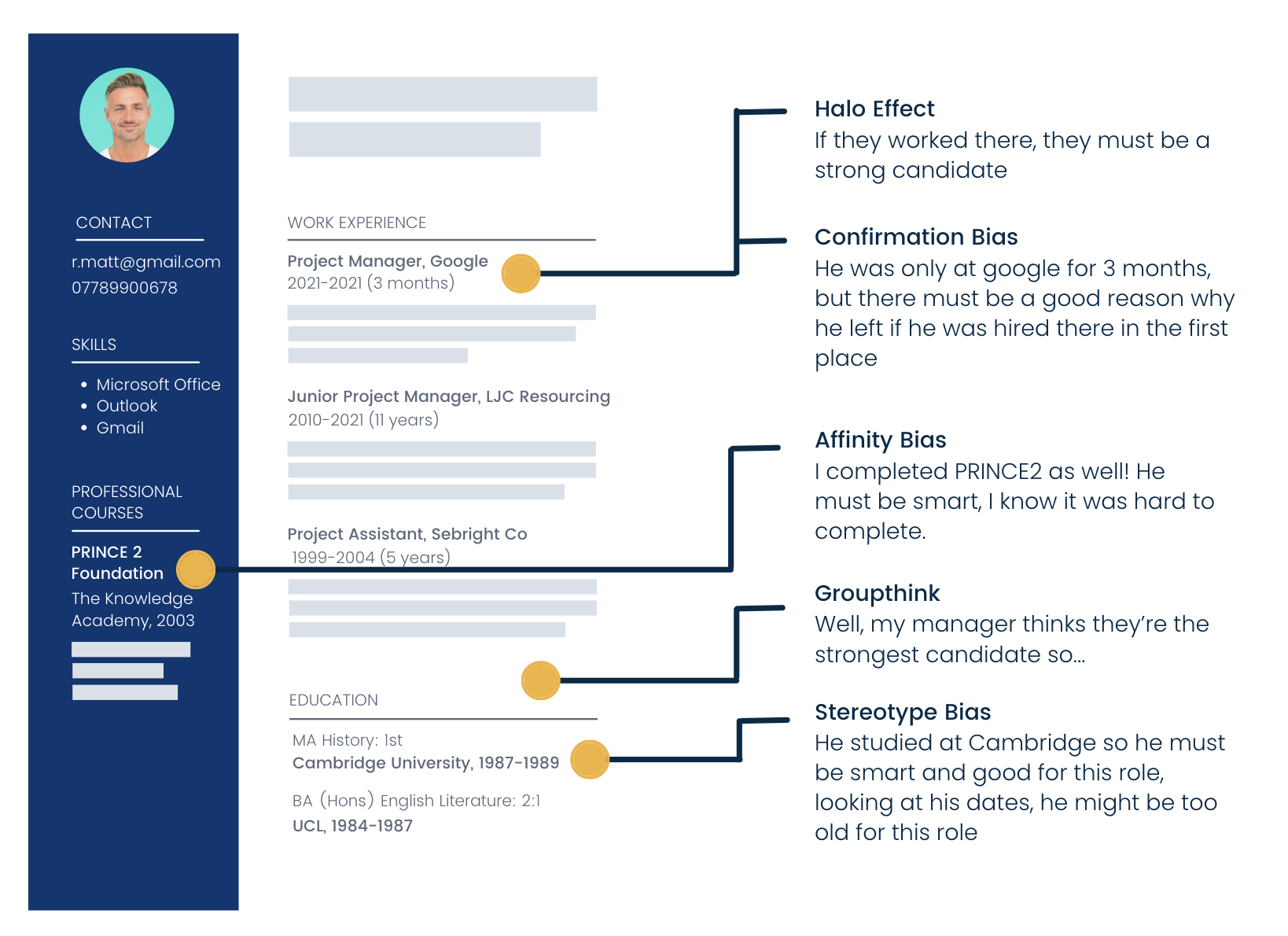
And even when we're made aware of them, outcomes still don't improve.
This is why unconscious bias training doesn't work... it's simply impossible to 'train' these biases away.
Our brains use two systems for decision-making:
- System 1: used for everyday, intuitive decisions like how much milk to pour into your morning coffee (a bit like being on autopilot).
- System 2: used for bigger, more important decisions like planning a project at work or a one-off trip.
System 1 is absolutely necessary in order for us to process the 1000s of insignificant micro-decisions we face every day. However, since it relies on mental shortcuts, shortcuts and past experience to jump to conclusions, this system of thinking can lead to harmful outcomes when it comes to people-based decisions.
Although we can't train biases out of humans, what we can do is design them out of our processes.
If you care about reducing bias and improving diversity - anonymise your screening!
Anonymisation works and won't cost you a penny.
Back in the 70s and 80s, orchestras were able to double the number of women making it through auditions just by doing them from behind a curtain.
And today we're able to boost ethnic diversity by 3-4x by removing identifying information from candidates' job applications.
Scoring rubric
Having objective, skill-based criteria to score candidates' answers against is best practice for both improving diversity and predictivity.
This doesn't need to be anything too elaborate, here at Applied we use a simple 1-5 star scale.
Here is the rubric we used to score the work sample above:
1 Star
- No real effort
- Launched into the sell without gathering information
3 Star
- Evidence of gathering relevant information about the
company/person
- Identifying a pain point,
- Clear follow-up plan
- Understanding that you are building a relationship, not trying
to close the deal.
5 Star
- Clear structure and sales process that prioritises gathering relevant information
around the person and wider commercial context to their
getting in touch.
- Emphasis on discovering their specific “Pain Point” and uncovering the
“critical event” i.e. the particular role or project that Applied
can be piloted on
- 5* answers will look to put a number or cost on the pain
- Success is a clear timeline for the next step, building rapport,
and successfully widening the conversation to include other
stakeholders in business
Diverse review panels
Crowd wisdom is the general rule that collective judgment is generally more accurate than that of an individual. And it's fairer too! Any individual reviewer's biases will be averaged out.
Research tells us that three is the magic number before you start to see diminishing returns.
A few rough and ready rules for effective review panels:
- Diverse panel: The more diverse your three-person review panel is, the more unbiased the final scores are likely to be.
- Independent scoring: Having a 'no peeking' rule will ensure scores haven't been influenced in any way.
- Quantifiable results: Wisdom of the Crowds works best when inputs are quantified and aggregated.
Is is ever okay to use CVs?
The research is clear: CVs are fairly ineffective at predicting skills and supporting diversity.
However... if you're hiring for a role beyond your expertise or a very senior role, sometimes you might feel they're necessary.
If you are going to be screening resumes, try adding structure to your reviewing process instead of using AI to pick out keywords - this will be far more predictive and significantly less prone to biases.
The Applied Platform uses the CV Scoring Tool to make standard resume reviewing as objective and structured as it can be.
By focusing on the things that are actually predictive of future job performance, you'll make better hires, with less bias.
So, give yourself a set list of questions to score candidates against.
- Has this person been promoted in previous roles?
- Do they have experience in a similar working environment?

FACT: Any candidate screening practices that revolve around CVs are going to be far from perfect.
But by having objective criteria to score against and anonymising candidates, you can begin to data-proof what is typically a biased, instinct-driven process.
The best person for the job isn't always who you think they are... so don't use AI to find them.
60% of people hired through our anonymous, skill-based assessments would've been overlooked via a traditional talent acquisition process.
Why? Because although skills are often honed whilst studying at a prestigious university or at a big-name organisation, it can also be an outsider perspective that makes someone the best person for the job.
Using an AI to draw patterns is always going to fall short... because without actually testing someone's skills there is no template for what 'good' looks like.
The overwhelming majority of data we have about who did or didn't get hired will have been influenced by human biases and socioeconomic inequalities.
Rather than levelling the playing field, AI recruitment software is more likely to just further handicap some players.
Not all recruitment automation is inherently sinister, but it should be used to reduce biases and uncover hidden talent rather than just to speed up an already biased and ineffective process.
Who are we? And what is Applied?
Born out of the UK Government's Behavioural Insights Team, Applied is essential recruiting software for fairer hiring.
We built Applied to help organisations source, assess and hire candidates using proven, research-backed assessments - automating all of the boring stuff so you can focus on identifying potential hires.
All of our core practices can be implemented without our platform - we gave away our entire process here.
What outcomes can you expect from using our process?
- Improved diversity: 3-4x more candidates from ethnic minority backgrounds
- Quality of hire: 3x more suitable candidates
- Better retention: 93% retention rate after year 1
- A process candidates genuinely enjoy: average 9/10 candidate experience rating
- Increased efficiency: 66% reduction in time spent hiring
If you want to take the next step and see how Applied works, you can explore the platform at your own pace here.

Applied is the all-in-one HR recruitment software purpose-built to reduce bias and reliably predict quality talent.
Start transforming your hiring now: book in a demo.