
Updated 06/12/21 with additional studies and best practices for reducing gender bias in the hiring process
DID YOU KNOW? According to a recent UN report, almost 90% of men and women hold some sort of bias against women.
Whilst some countries are doing better than others, there are no countries in the world with true gender equality, the study found.
Most hirers understand that gender equality is better for business as well as simply being the right thing to do…
But even the most well-intentioned advocates of diversity are prone to bias.
Unconscious bias training doesn’t work.
However, there are solid, evidence-based steps you can take to de-bias your hiring process to attract/ hire more women...
Gender bias in hiring: the report
- The state of play
- The science behind unconscious bias
- Why bias training doesn’t work
- Strategies for improving gender equality
- Why is gender equality important?
Gender bias in hiring statistics - the state of play
Women have increased their presence in senior management and leadership roles over time but remain under-represented in top company positions.
Whilst society may seem to be getting more progressive, gender equality progress is actually slowing down over time.

Make no mistake - this is not a problem that is going to ‘sort itself out’ or gradually improve over time unless we take action.
Gender discrimination in hiring (UK)
A UK study (looking primarily at the effect of social class indicators) found that a candidate’s gender had a measurable impact on callback rates - albeit not an extreme one.

Here you can see that men were called back in slightly higher numbers than women.
However, this doesn’t tell the full story.
To get the full picture, you have to drill down into individual roles.
Below are the results of a study carried out across the English labour market back in 2006.
Researchers sent carefully-matched applications to open job vacancies, specifically testing for gender discrimination in hiring.
Leaving everything else the same, they changed only the gender on the application.
Here are the results:
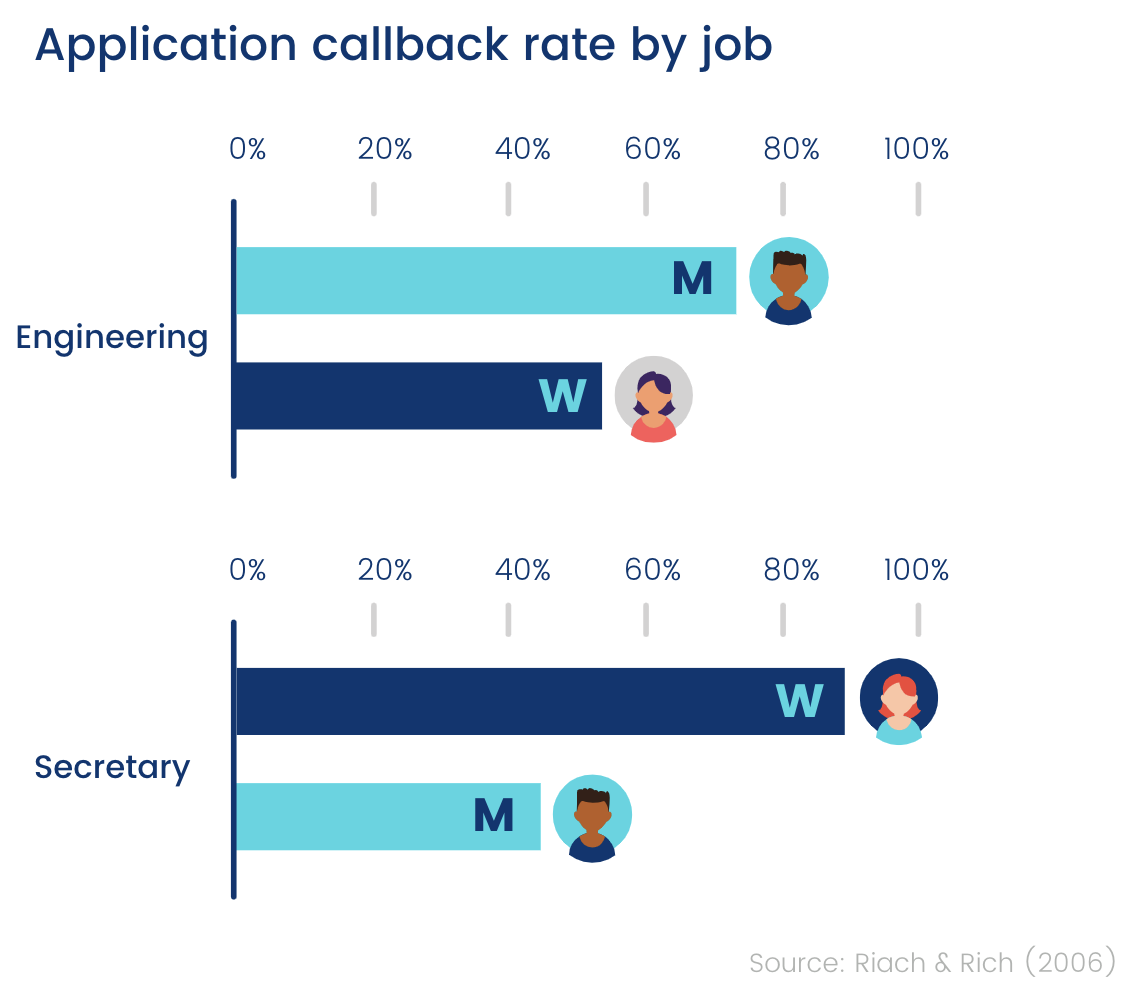
What does this tell us?
Well, it tells us that men tend to be favoured for typically male roles.
And women tend to be favoured for typically female roles.
If a job is dominated by the opposite sex, you’re likely to be penalized.
So, if top positions are generally male-dominated, women will find it harder to get into these positions.
And just a quick look at the FTSE 100 confirms this:
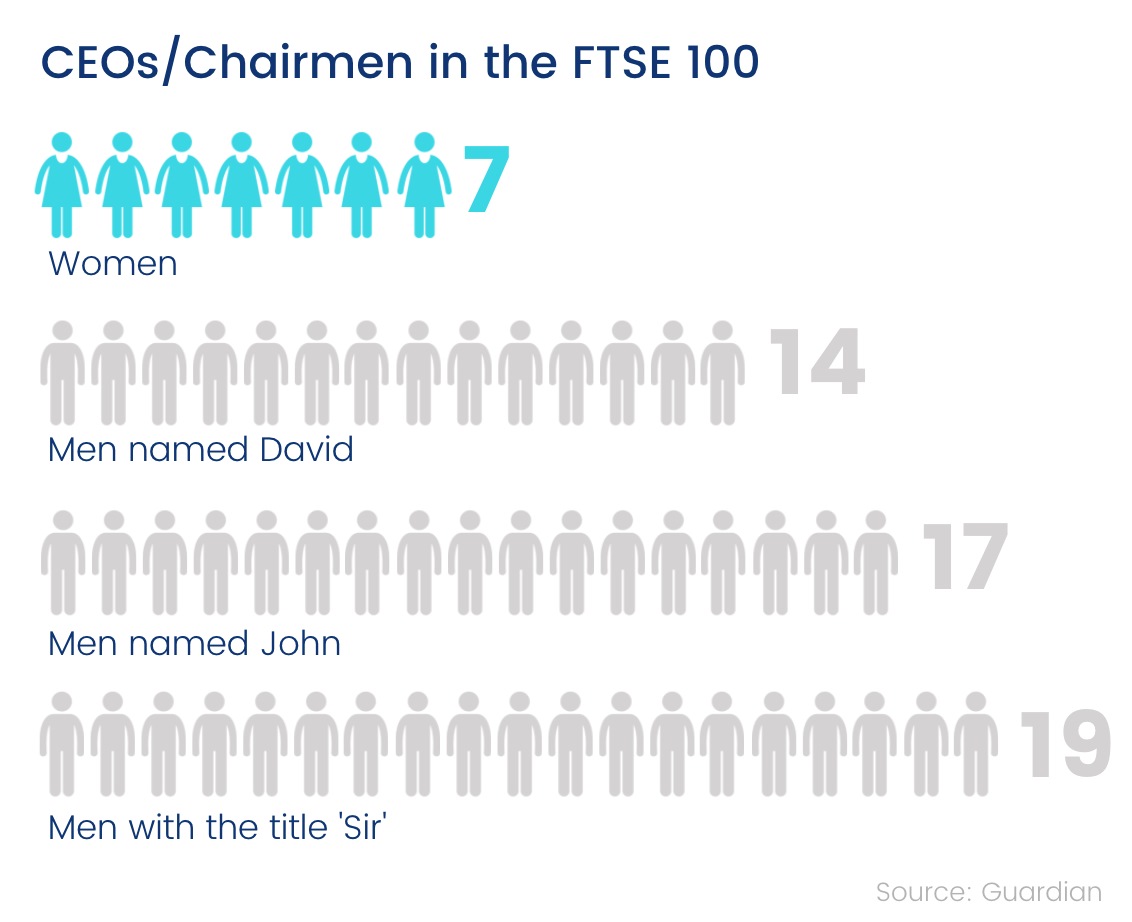
In the FTSE 100, there are more CEOs/ chairmen called John than there are women.
Gender discrimination in hiring (USA)
Despite efforts to hire more women, academic science suffers from a significant gender disparity.
Suspecting that this may be due to a gender bias in hiring, U.S researchers had the science faculties of research-intensive universities rate candidates' applications to a laboratory manager position.
Applications were randomly assigned either a typically male or female name.
Candidates were rated in terms of competence, hireability, and likelihood of receiving mentorship:
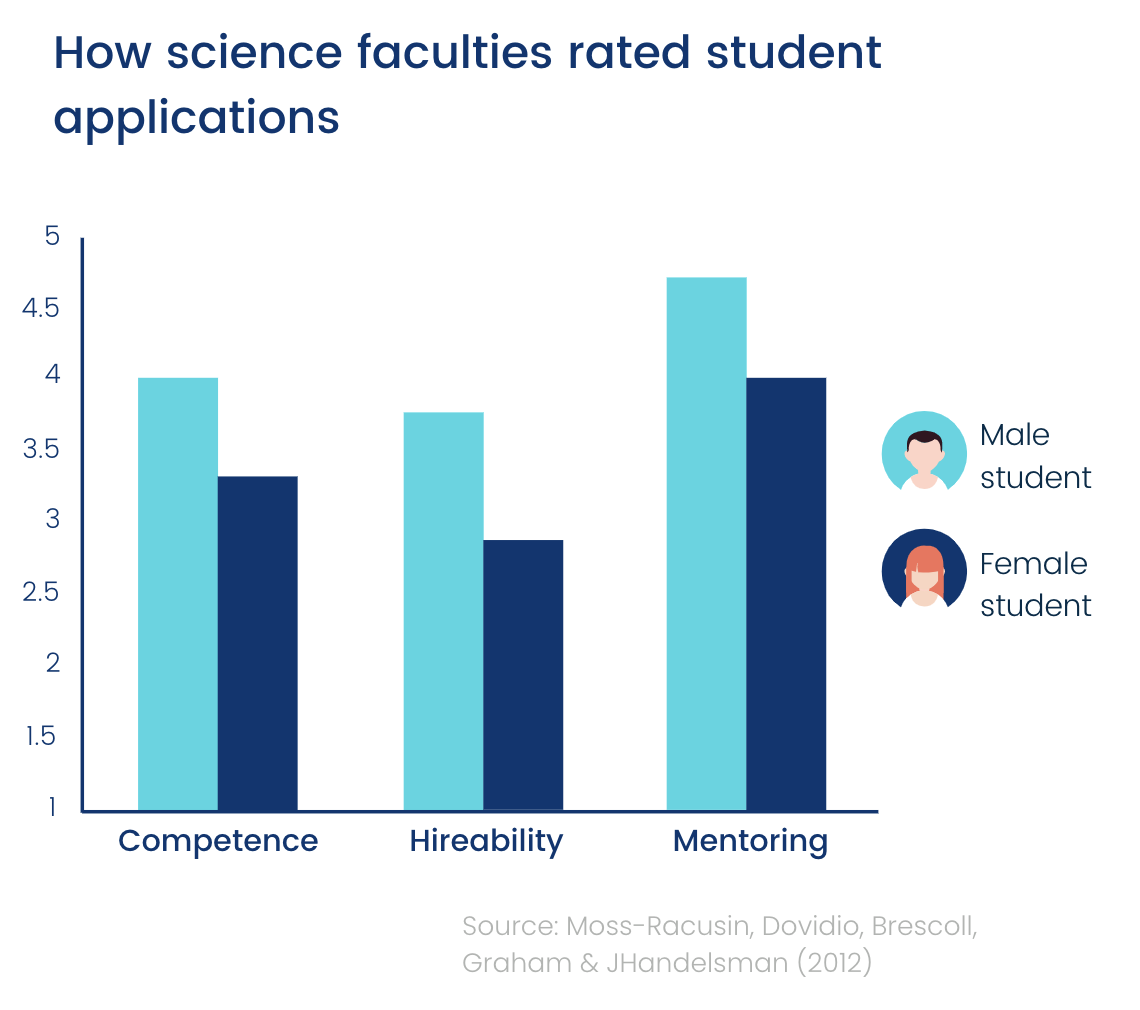
The study found that participants rated women as being less competent and hireable than men - despite their applications being identical.
Men were even offered higher starting salaries.

Although you might automatically assume that this is a result of a male bias against women, this wasn’t the case.
The gender of the hirer did not affect responses.
Female and male faculty were equally likely to be biased against women.
In another study, this time looking at class and gender bias in hiring, researchers sent applications to elite jobs at U.S law firms.
They created two fictitious candidates, matched on all characteristics except gender and class indicators.
Here’s what they found:
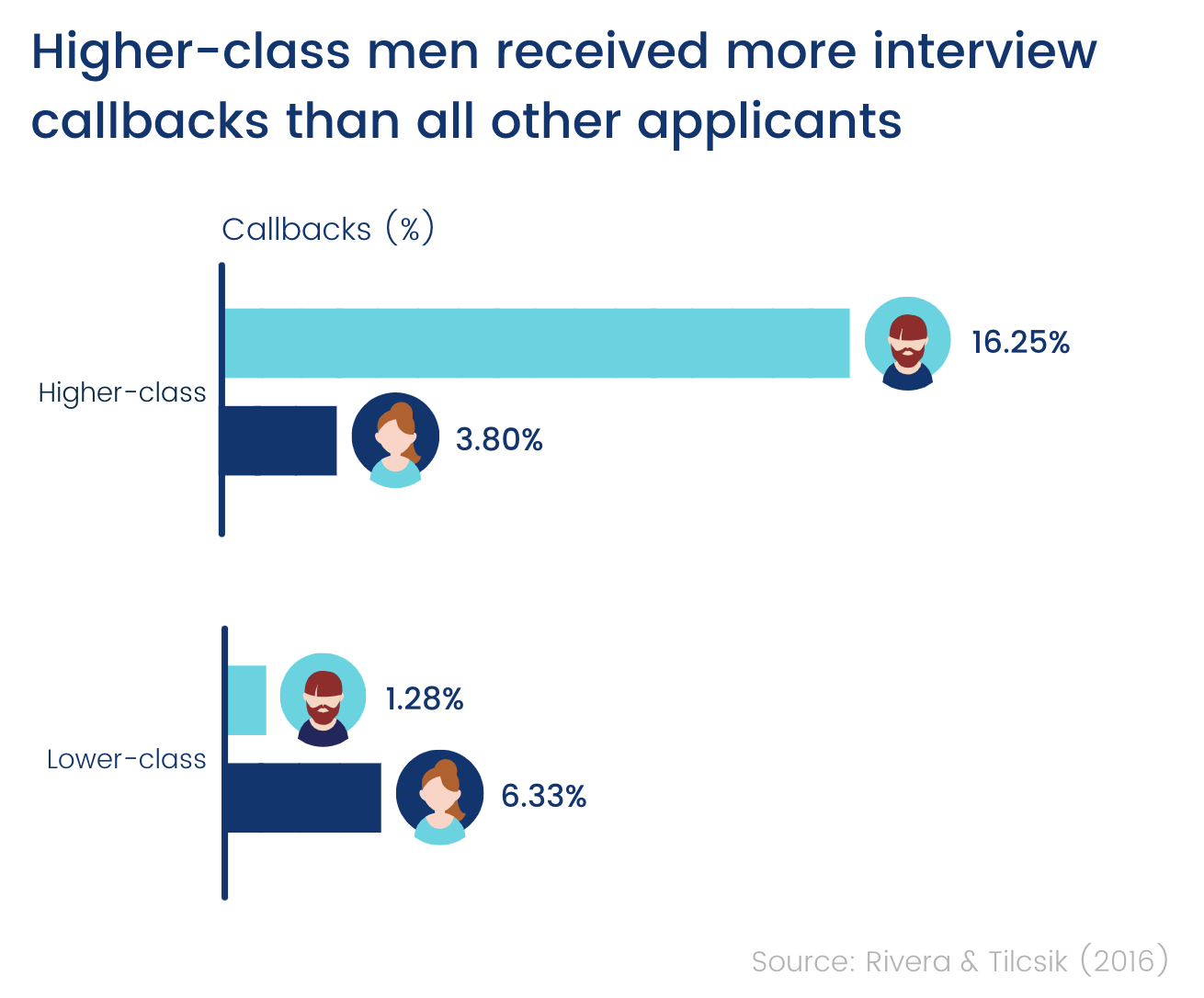
The study concluded that only men perceived as higher class had an advantage when applying to the most elite jobs.
For women, a higher-class background actually led to a “commitment penalty.”
Interviews with the employers found that they viewed higher-class women as being less committed to working and more likely to leave after having children.
This applied across all candidates, regardless of their actual parental status.
Gender discrimination in hiring (EU)
A Eurobarometer survey released back in 2017, emphasised that gender equality has still not been achieved in the EU Member States.
If we look at the research, this seems to ring true.
A recent study, conducted in 2020, looked at gender bias in hiring for two male-dominated professions - mechanics and IT.
Recruiters for real-life open roles across Bulgaria, Greece, Norway and Switzerland were asked to rate hypothetical CVs.
The CVs were identical, except for the gender of the applicant.
Below are the results - anything below the grey line represents discrimination.
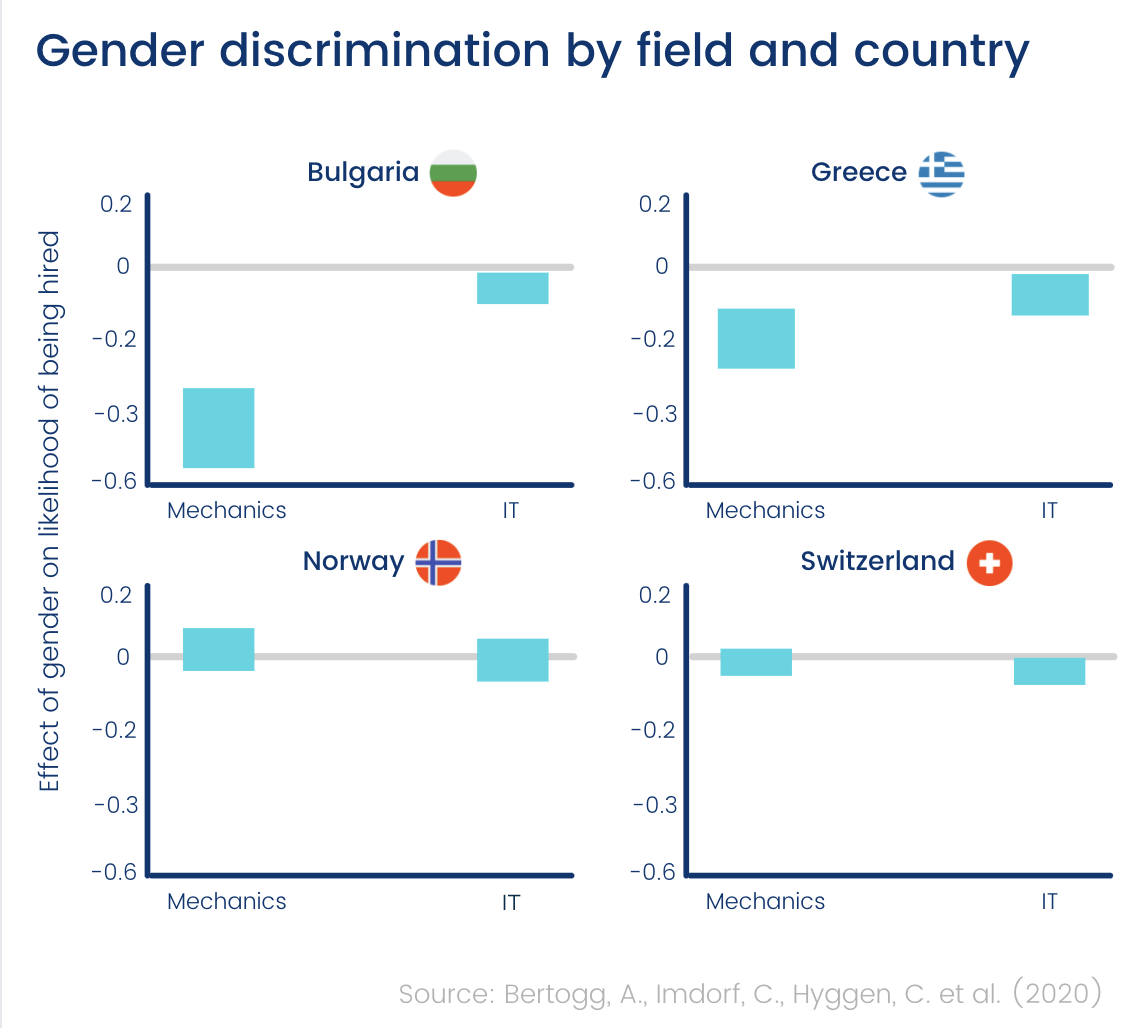
As you can see from the charts above, women have a lower likelihood of being hired in…
- Bulgaria (female mechanics were rated 43% less positively than males)
- Greece (female mechanics were rated about 18% less positively than males)
- Switzerland (female IT candidates were rated around 5% less positively than males)
Aside from just competence, women have also been found to suffer from parenthood-based discrimination.
Researchers investigating gender equality in Spain set out to determine how much of an affect being a parent had on hiring success.
CVs were sent in response to 1,372 job ads for a variety of roles.
Take a look at the results below:

The researchers found that not only was there a general gender bias in hiring, but being a parent had a significantly more detrimental effect on women than men.
This isn’t just a Spanish phenomena, studies in the U.S have had similar findings...
- 'Highly successful mothers tend to be discriminated against in hiring and promoting decisions because they are viewed as less warm, less likeable and more interpersonally hostile' (see study).
- 'Mothers face penalties in hiring, starting salaries and perceived competence, while fathers can benefit from being a parent: for women, competency ratings were 10% lower for mothers compared to non-mothers among otherwise equal candidates' (see study).
What about AI?
In a recent Australian study, researchers gave 40 recruiters real-life CVs for jobs at UniBank.
The CVs were identical, except half had the candidate’s gender, and the other half were assigned either (traditionally) male or female names.
The recruiters were then asked to rank each candidate and collectively pick the top and bottom three CVs for each role.
Here’s how they ranked the resumes…

Recruiters preferred CVs from male candidates - even though their CVs stated exactly the same education and experience.
Both male and female panelists showed this bias.
The researchers used this data to create a hiring algorithm for ranking each candidate.
Unsurprisingly, they found that it reflected the recruiters' biases.
If the data being used to ‘train’ the AI was based on a biased data set, was it any wonder that the algorithm itself was also biased?
The results of this experiment can also be seen in real-life...
Amazon’s recruitment algorithm was found to display gender biases by downgrading CVs containing the word “women” or which contained reference to women’s colleges/ universities.
As was the case in the study above, the process of using ‘training data’ to build recommendations is often where the issue lies.
If the data is not objective then the algorithm will follow suit.
If you wanted to build an AI that finds the best candidates, you’d first need to identify what current 'best candidates' look like.
Let’s say you wanted to find a marketing or sales director - since around 66% of marketing and sales directors here in the UK are men, your algorithm would assume that a male name is a desirable quality for the position.
So, in a nutshell, AI is trained using human-made data sets. Humans are biased. As a result, the AI algorithms we build are also going to be biased.
Further reading around AI:
- Made by Humans by Ellen Broad
- Weapons of Math Destruction by Cathy O’Neil
- Algorithms of Oppression by Safiya Umoja Noble
- Applied- Why we don’t use AI for hiring decisions
The science behind unconscious bias: a brief overview
We’re all prone to unconscious bias.
This bias - so long as it’s unconscious - doesn’t make you a bad person.
It just means that you’re human.
We naturally tend to put others into categories based on physical appearance and basic background information.
How does unconscious bias happen?
We make 1000’s of decisions every day.
Some big.
Some small.
To manage this demand, our brain has two ‘systems’ for decision-making (a theory popularized by Daniel Kahneman’s book, Thinking Fast and Slow).
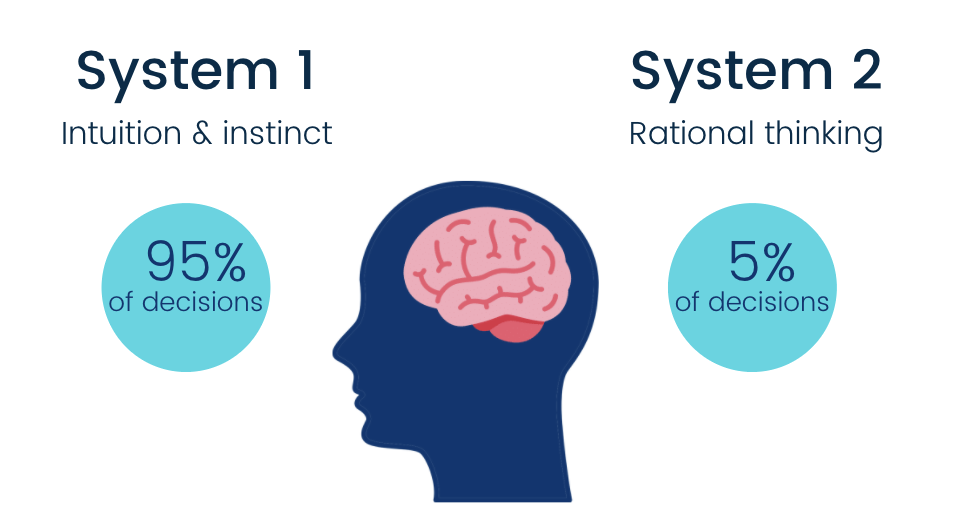
- System 1: for fast, intuitive thinking
- System 2: for slower, more conscious thinking
System 1 is what we use to handle all of the micro-decisions that we make each day.
This is how you might find yourself walking to work on ‘auto-pilot.’
This system of thinking relies on mental-shortcuts and associations.
You see a red light, you instinctively stop.
You hear a familiar voice, you turn around.
Without System 1, we wouldn't be able to function on a daily basis.
We have so many micro-decisions to make that we can’t stop to ponder each one equally.
System 2, on the other hand, is for making those less frequent, more important decisions.
When planning a big project at work, you’d be using System 2 (you’d hope!).
If you were going to make a big purchase, you’d also be using System 2.
These are decisions that aren’t made using ‘gut instinct’ - they require a conscious effort.
gender bias in the workplace tends to occur when we fall back on using System 1, since it relies on subconscious shortcuts and a ‘gut instinct’ of sorts.
This isn’t something intentional.
We do this without even realising.
Our brains use shortcuts and patterns to draw conclusions, by using the information subconsciously stored in our mental lockers: this includes things like our past experiences, what we’ve seen in the media and our upbringing.
Whilst this is necessary to help us make sense of our complicated world, it can result in harmful outcomes when it comes to decisions involving people.
Stereotypes dictate who we expect to see in a given role
Stereotypes act as a rule of thumb in terms of what is possible.
This is why women find it easier to get jobs as secretaries and men as engineers.
Generally, it’s not a case of men intentionally trying to keep women out of a given profession (although this may sometimes be the case), it’s more about the kind of person we expect to see in a role.
Whether you yourself are a man or woman, you’ll typically associate certain groups with certain characteristics and roles.
We might think of surgeons as being men aged 30-50.
We might think of nurses as being women aged 20-40.
These stereotypes are bred into us as children and continually perpetuated throughout our adult lives.
When it comes to hiring for these roles, we might subconsciously look for someone who ‘fits the bill.’
In other words, we’ll look for someone who fits the stereotype of someone in that role.
Not conforming to a stereotype tends to result in penalization
Looking at gender bias in hiring at a high level, it may seem that discrimination is minimal.
And in some studies, women were even called back at higher rates than men.
However, you don't have to scratch very deeply beneath the surface to discover that such discrimination does in fact exist.
If women apply for ‘typically female’ roles, then their chances of a callback are fairly high.
So no, they aren’t subject to hiring discrimination…
As long as they ‘stay in their lane’.
On the whole, senior positions pay more than junior/mid-level positions.
And according to Mercer’s 2020 report, the higher up the corporate ladder you go, the fewer women you’ll come across.
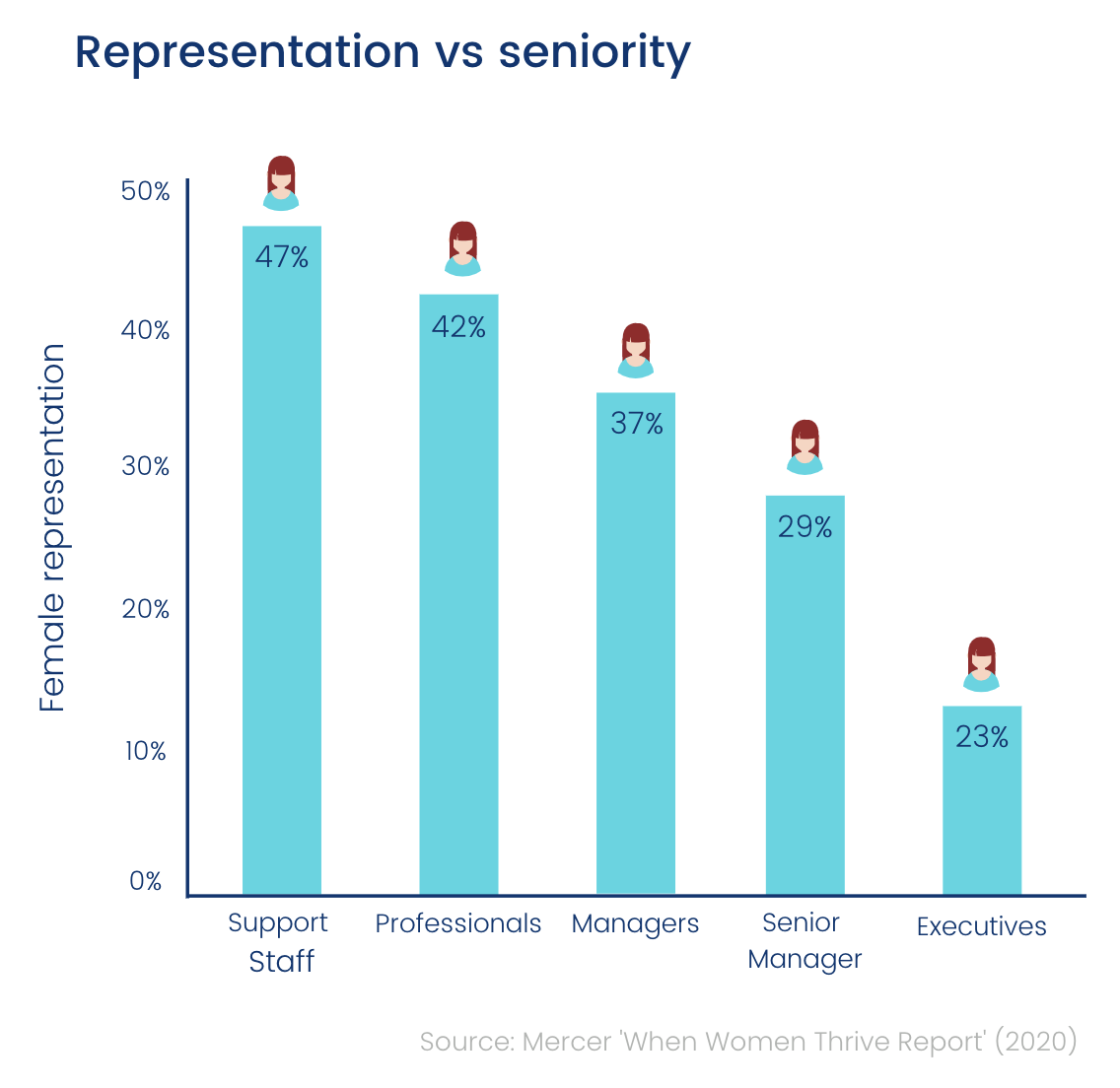
So whilst women may be able to get some jobs easily enough...
They can’t necessarily get the best paying ones with the same level of ease as their male counterparts.
Even when you remove seniority from the equation and just look at job functions, women are over-represented in support functions like administration, while men generally dominate operations, profit/loss and research.
Note: these three male-dominated disciplines are viewed as ‘critical’ experiences for CEOs and board members to have.
This ‘subversion tax’ even applies to how womens' skills and general demeanour are perceived.
Attributes we may praise when exhibited by men tend to be seen as negative when exhibited by women.
The most famous example of this is the Heidi Roizen case study.
Heidi Roizen was a successful Silicon Valley venture capitalist.
At Columbia Business School, half of a class was presented with her case study with her real name on it (Heidi).
The other half had the same case study, except the name was changed to ‘Howard’.
The students rated ‘Howard’ and Heidi as equally competent…
But they would have preferred to work with Howard.
Heidi was also perceived as being significantly less likeable and worthy of being hired than Howard, as well as more selfish.
Further studies and surveys also confirm that we have set ideas around the roles men/women should and shouldn't be doing.
According to a European Commission survey, although 84% of participants believed that gender equality was important (including 80% of men), the survey also showed that more than one third of Europeans think that men are more ambitious than women (35%).
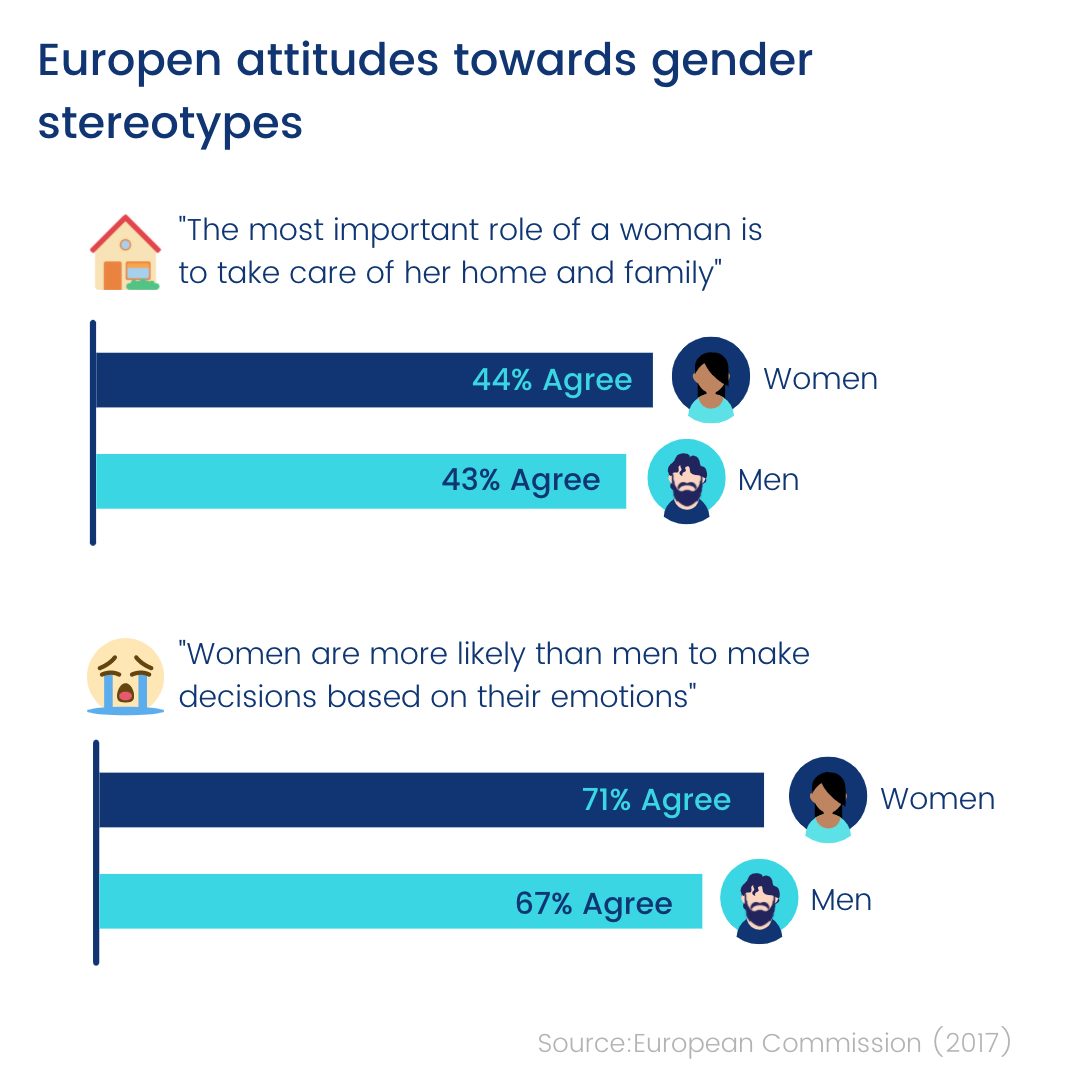
Why unconscious bias training doesn’t work - a quick look at the evidence
Although the diversity training market is valued at around nearly $8 billion annually, research tells us that it’s simply not worth investing time and money into.
One of the most positive studies of bias training discovered that it heightens people's ability to detect the gender diversity of an environment. Those involved in the training were then more sensitive to the diversity balance of their team and the wider organization.
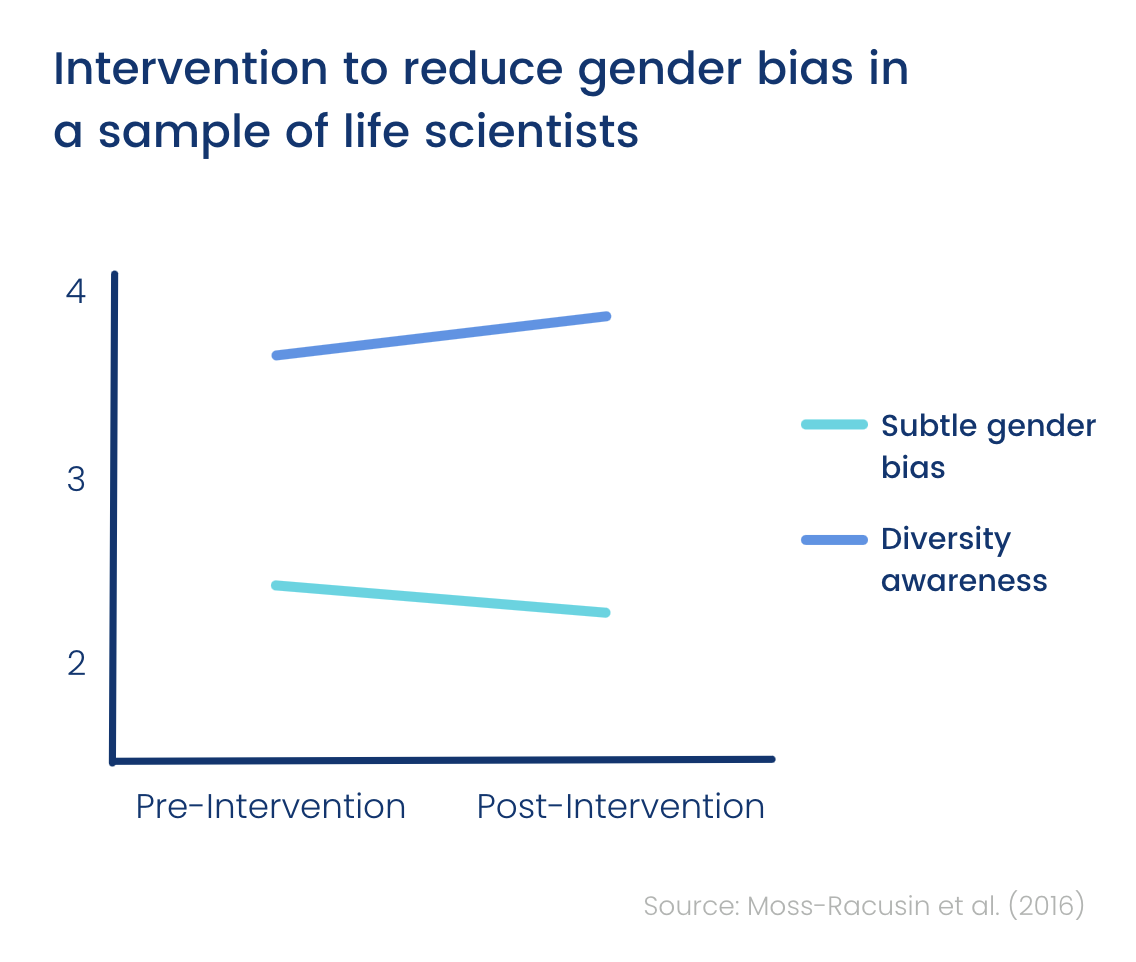
However, whilst effective at raising awareness, training doesn't actually reduce bias...
A meta-analysis of 426 studies found that while there was a reduction in bias immediately after training, this disappeared after about 8 weeks.
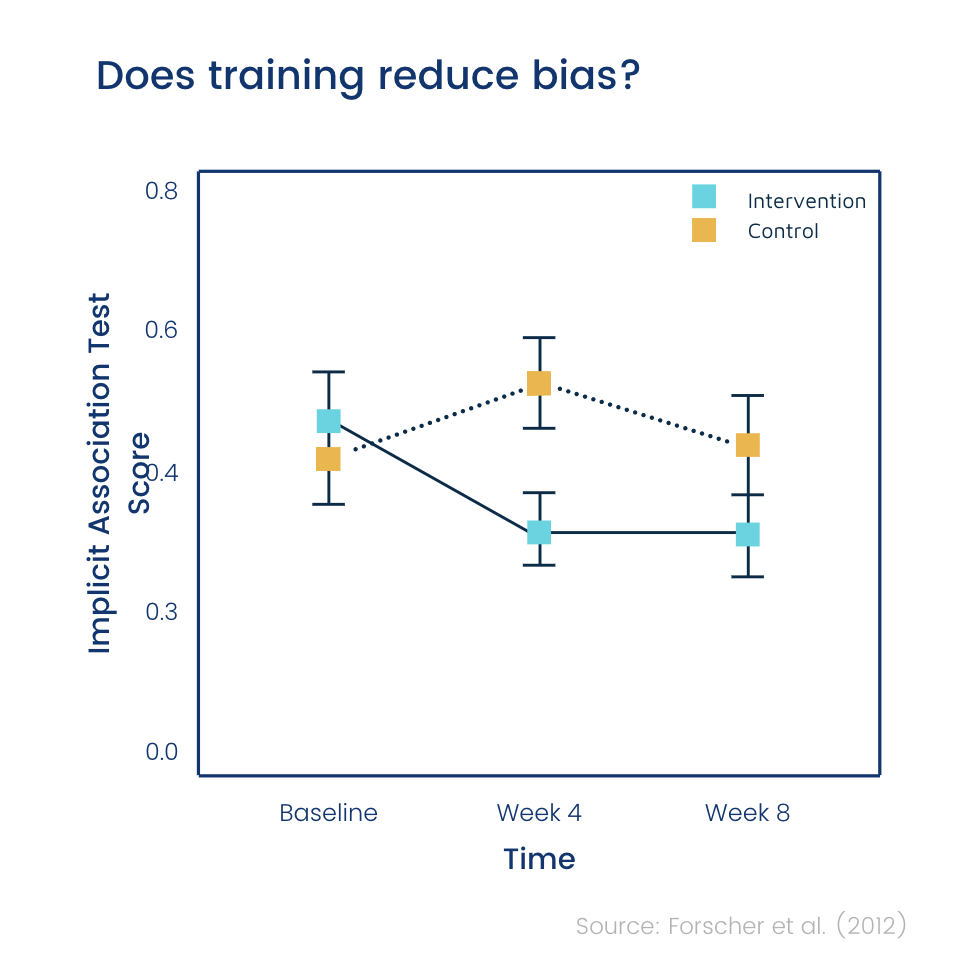
When it comes to changing behaviour, and impacting real-world diversity, a study of 829 companies over 31 years showed that training had no positive effects in the average workplace.
A more rigorous study of unconscious bias training delivered an hour-long diversity course to thousands of employees at an international professional services company.
Participants reported improved acknowledgement of their own biases and greater support of women in the workplace.
However, this didn’t translate into lasting behavioural change.
Three weeks after taking the course, participants were no more likely to take on a female mentee.
And six weeks after taking the course, participants were also found to be no more likely to nominate a female colleague for recognition of their “excellence”.
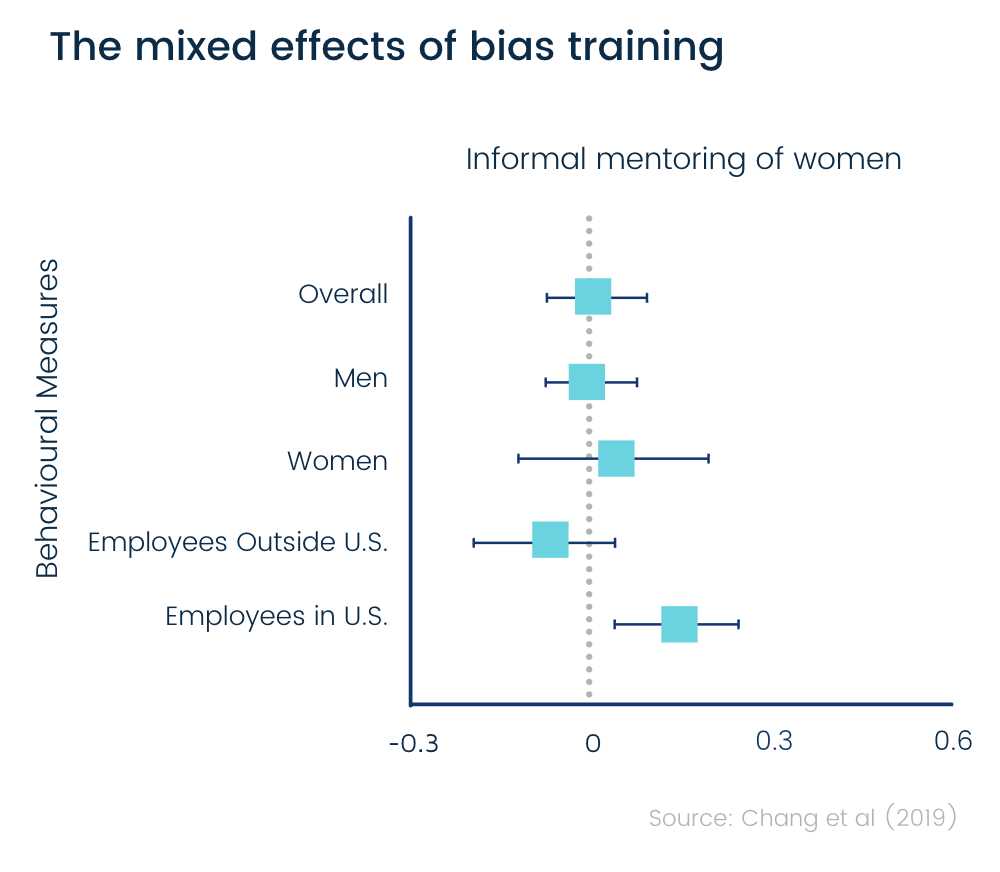
So, it's not that bias training is completely useless.
It does have some effect on bias.
It’s just that it’s extremely expensive and there are far cheaper means of reducing bias that will have a greater, longer lasting impact.
Bias training doesn’t work because it attempts to change what is essentially human nature.
Most of us are largely unaware of our biases, and so even when we’re made aware of them, we cannot rid ourselves of bias - no matter how well-intentioned we may be.
Although you can’t debias people, you can de-bias environments and processes.
Rather than changing how people themselves make choices, behavioural scientists have found that redesigning the environment in which people make choices has a far more significant impact on real-world outcomes .
The most famous example of this involves orchestra auditions.
In an attempt to improve the gender diversity of orchestras, behavioural scientists set up ‘blind’ auditions - which had candidates perform behind a curtain.
As a result, double the number of women got through the auditions.
The scientist knew they couldn’t remove bias from the panel themselves, but they could remove bias from the audition process.
This same principle has been proven to work for debiasing hiring too.
You can’t train hirers to be less biased.
But you can reduce the bias in your hiring process - without barely spending a penny - by simply designing an environment that makes bias less likely.
Gender bias in hiring: strategies for improving gender equality
Here at Applied, we're on a mission to change how the world hires.
By designing a process that mitigates bias and focussing solely on the skills needed for the job, we can improve diversity without the legal and ethical baggage that comes with positive action and diversity quotas.
We know this process works - just take look at tech hires through our platform...
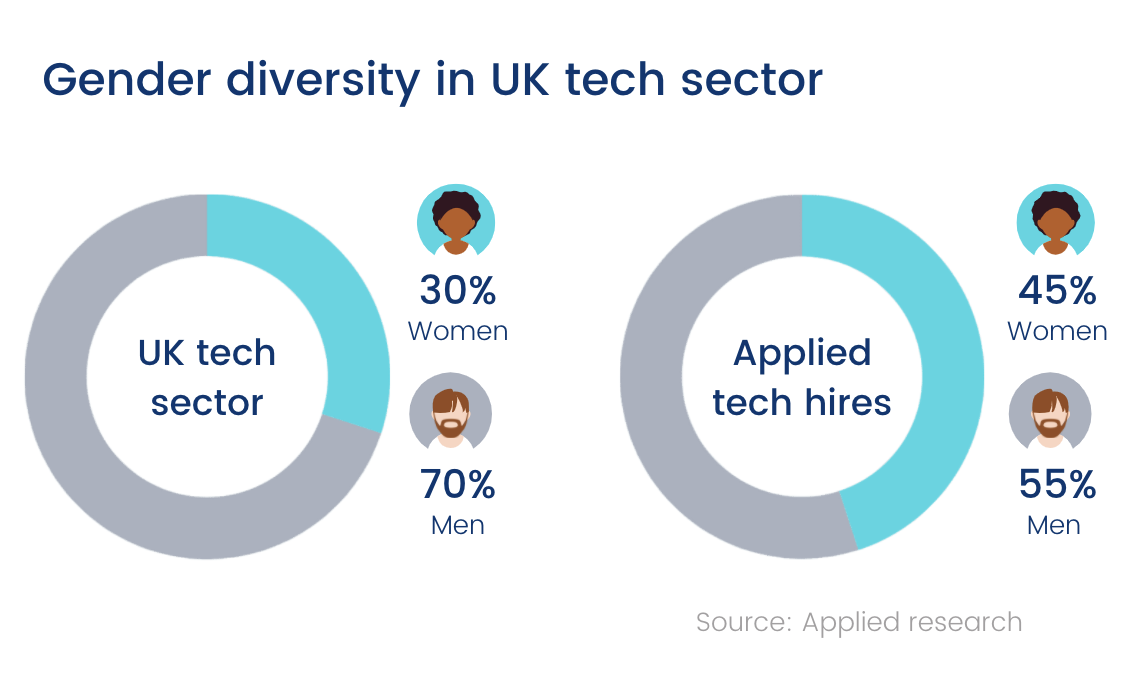
Sourcing
Sourcing can be a red herring when it comes to improving diversity.
Organizations often assume their diversity issues can be solved simply by attracting a more diverse set of candidates at the very top of the funnel, when in reality, the rest of their process is also harming diversity as a result of bias.
However, if you’re struggling to source enough women, there are some practical, evidence-based steps you can take to address this.
Write inclusive job descriptions
The language you use in your job description will have a direct impact on who actually applies.
Certain words and phrases can convey subconscious meaning that signals to candidates whether or not they’d belong in your workplace.
Women are put off applying if the job description includes excessive ‘masculine language’ (or ‘masculine-coded’ language).
Below are a few examples of gender-coded language commonly used in job descriptions:
Masculine terms:
- Analytical
- Autonomous
- Decisive
- Independent
- Leader
- Self-sufficient
Feminine terms:
- Committed
- Dependable
- Honest
- Loyal
- Supportive
- Trustworthy
By requiring characteristics typically attributed to men, you’re signalling that a man would be a better fit.
Whilst the effect of gendered language will vary from person to person, using masculine language will have an overall impact on the gender diversity of your candidate pool.
We looked at the effect of gender-coded language on gender diversity, using data from our Job Description Analysis Tool:

- 54% female applicants when job description was strongly feminine
- 51% female applicants when job description was feminine
- 48% female applicants when job description was masculine
- 44% female applicants when job description was strongly masculine
Key takeaway: feminine-coded job descriptions will increase the odds of women applying, while masculine-coded job descriptions will discourage women.
We then looked at the diversity of the people who were actually hired based on these job descriptions:

Again, we can see that feminine-coded job descriptions increase the odds of hiring a woman, and strongly masculine-coded job descriptions reduce those odds.
Aside from gendered language, how you list your requirements can also lead to gender bias in your hiring process.
Research has shown that generally speaking, women tend not to apply for roles unless they meet 100% of the criteria, whereas men will apply when they meet just 60% of the requirements.
Why does this happen?
It’s likely due to a combination of gendered differences in confidence.
Women are more socialised to follow the rules and men are socialised to believe rules are meant to be broken.
If you list too many ‘requirements’ - women are more likely to perceive them as actual requirements they must fulfil in order to apply.
And men will be more likely to perceive them as mere desirables.
Note: This risk-aversion doesn’t make someone less capable of doing the given job. Being risk-averse has nothing to do with ability or competence.
Key takeaway: Only list requirements that are essential, no more than ten.
Job boards
Not all job boards are going to bring you the same degree of diversity.
Some will bring more women than men and vice versa.
Given that a single job can cost £100 - £400, you’ll want to make sure your postings are attracting a diverse candidate pool.
The easiest (and cheapest) way to do this is by using UTM links.
By providing each job board you post to with a unique, trackable link, you can monitor where your candidates are coming from and distribute your budget accordingly.
To help get you started, we broke down the best job boards for gender diversity here.
If you’re still struggling to attract an even gender split (which may be the case for typically male-dominated roles), you can also try posting to specialist, women-only job boards.
Specialist job boards:
Key takeaway: start tracking your job boards to identify which ones bring the most diverse set of candidates
Screening
Screening is where most of the gender bias in hiring occurs, so if you only have the time/ resources to change one part of your process, make sure it's this stage.
You can source more female candidates into your initial candidate pool but if your screening process isn’t fair and objective, your sourcing efforts will have been in vain.
Anonymize applications and ditch CVs
Most of the research around gender bias in hiring uses the names on CVs to determine the degree of bias.
So, the most logical step to prevent this bias is to simply remove names from applications altogether (as well as address, date of birth etc).
Anonymous applications with identifying information removed are undoubtedly a step in the right direction towards gender equality (at least in terms of hiring).
However, even with this information removed, what’s left still leads to bias. If we look at a standard CV, we see a candidate's work experience (usually with some details around what the candidate achieved in each role) and their academic background.
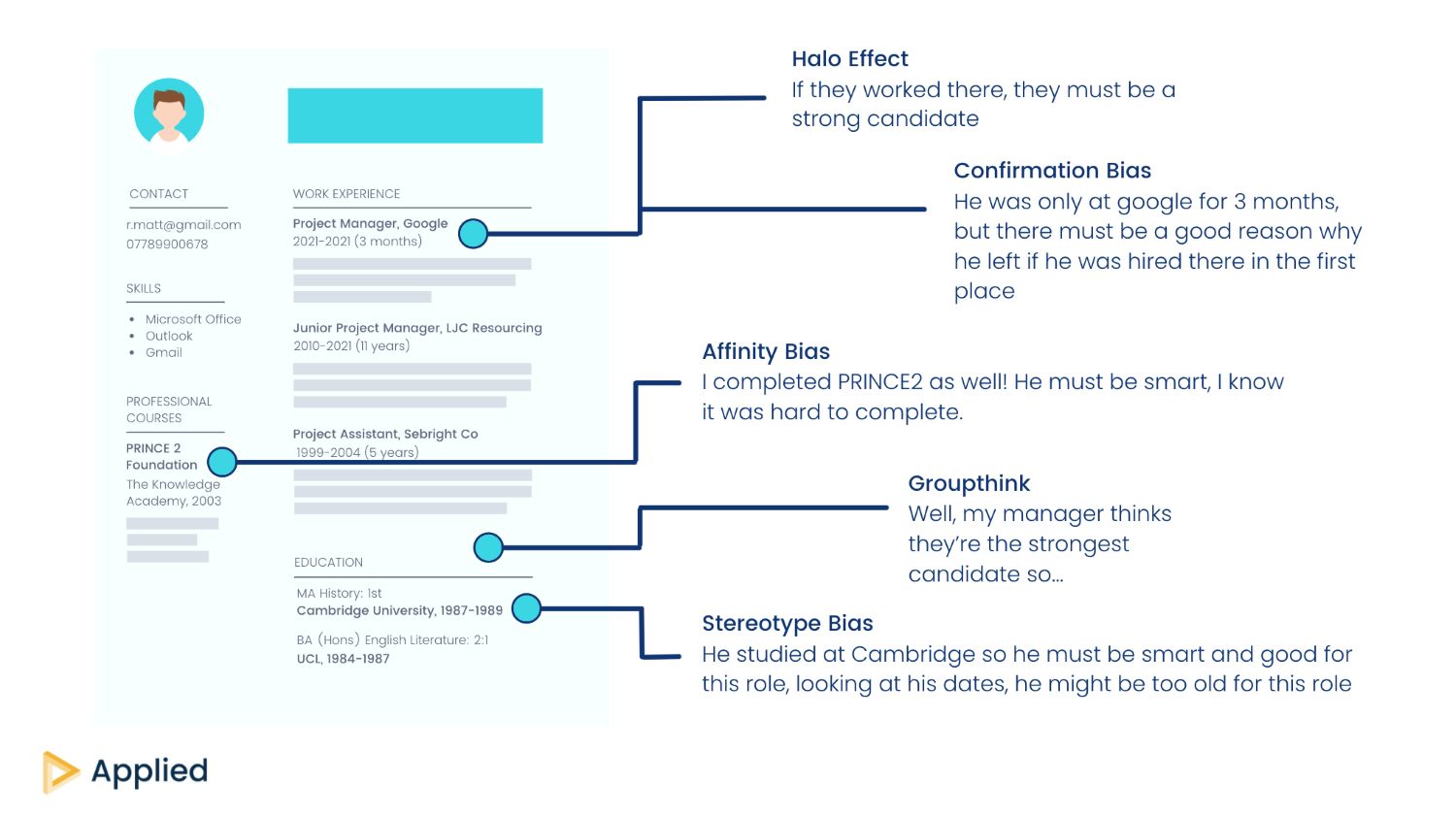
If we look at a standard CV, we see a candidate's work experience (usually with some details around what the candidate achieved in each role) and their academic background.
Although these may seem like fairly solid indicators of ability, the evidence suggests otherwise.
Firstly, even when we remove names (which indicate gender) from CVs, there’s still the fact that women have a tendency to downplay their achievements.
On average, women rate their performance less favourably than equally performing men.
And secondly, proxies like education and experience just aren't very good predictors of job performance.
According to the Schmidt-Hunter meta-analysis, education and experience don’t actually tell us much about a candidate's real-life ability.
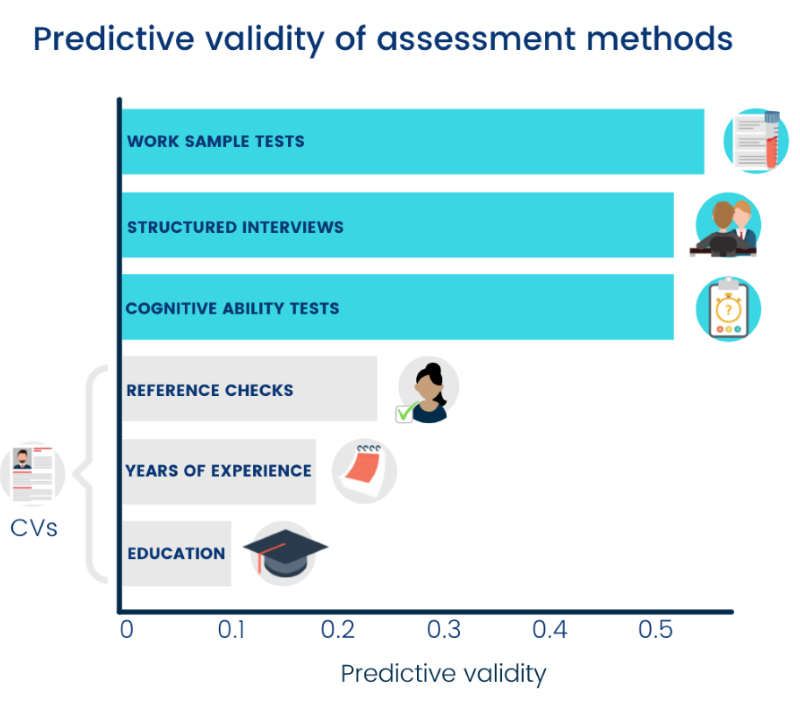
Popular assessment methods were mapped against their predictive validity (how accurately they predict future job performance), and as you can see, years of experience and education - staples of the CV - were found to be the least predictive means of assessment.
So if CVs result in bias and aren’t predictive, what should you use instead?
Looking back at the chart above, we can see that ‘work sample tests’ are the most predictive assessment method.
Although the Schmidt-Hunter study looked specifically at manual labour, we decided to adapt the work sample test for office-based jobs in order to accurately identify the best person for any given job.
Key takeaway: Remove identifying information from applications to prevent biases from being triggered
What are work samples?
Work samples are interview-style questions designed to the skills required for the job.
They work by taking a realistic task or scenario that candidates would encounter in the role and asking them to either perform the task or explain how they would go about doing so.
The idea is to simulate the role as closely as possible by having candidates perform small parts of it.
Instead of candidates talking through their achievements or why they’d be a great hire, work samples directly test the relevant skills.
Given that females are less inclined to self-promote, work samples level the playing field by removing the need to ‘talk up’ skills/ achievements.
Candidates aren’t required to talk about their skills, they’re instead asked to demonstrate their skills.
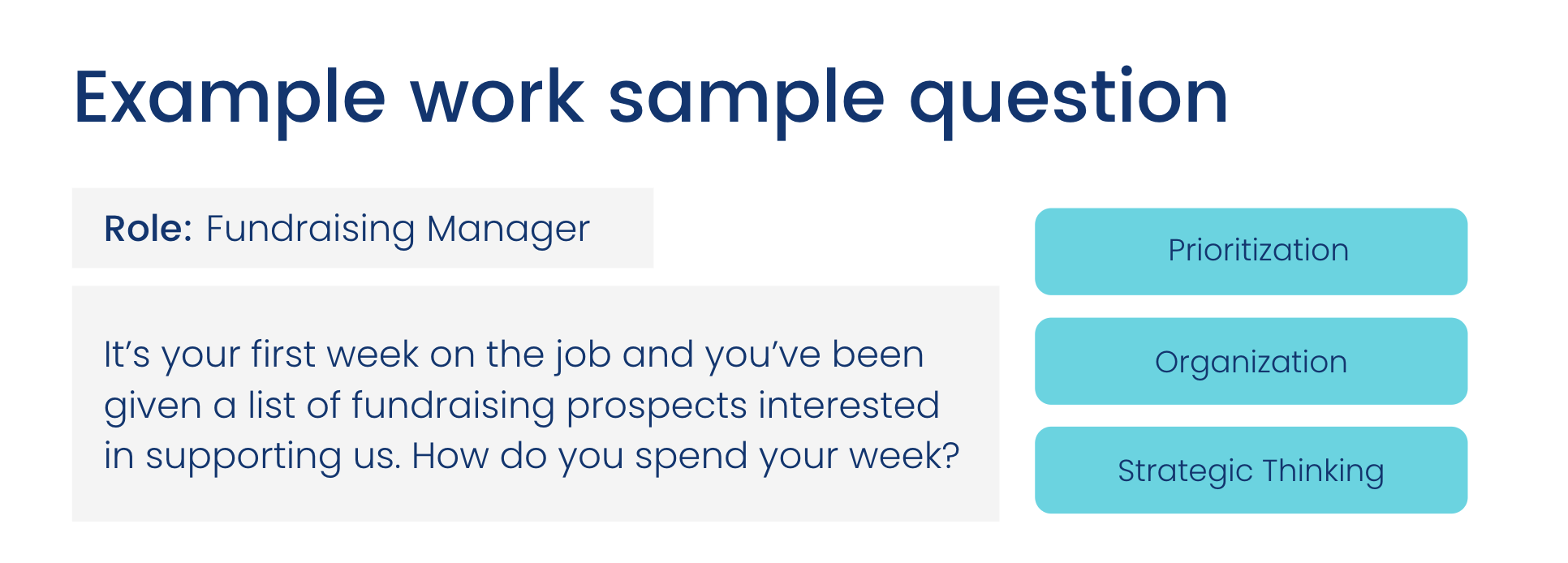
The more closely your work samples mirror real-life, the more predictive they’ll be.
The scenario above is one that the candidate would undoubtedly have to tackle if they were to get the job.
How to create your own work samples:
- Decide on essential 6-8 skills necessary to succeed in the role.
- Think of a job-specific scenario or task that would require 1-3 of these skills. This could be a day-to-day recurring task or a one-off issue. You could think back to a scenario that has already happened, or one that is likely to happen in the future.
- Once you have 3-5 tasks/ scenarios, turn them into work samples by posing them hypothetically.
- Give yourself scoring criteria - ideally a 1-5 star scale with a few bullet points for each star.
Work sample ideas:
- Email to potential lead
- Blog post draft
- Customer complaint to tackle
- Prioritization task
- Analysis of favourite website/ product/ service etc
- Logistical problem that needs to be solved
-
You can grab our (free) Work Sample Cheatsheet to hit the ground running - we collected some of the work samples we used to hire the Applied Team!
Key takeaway: Instead of CVs, have candidates answer 3-5 work sample questions anonymously.
Interviewing
When meeting candidates face-to-face, you’re inevitably going to be subject to some degree of unconscious bias.
According to one study, roughly 5% of interview decisions were made within the first minute of the interview, and nearly 30% within five minutes.
Another study found that candidates have around six minutes and 25 seconds to impress potential employers during their first meeting.
More often than not, hastily-made decisions based on a ‘feeling’ use the System 1 part of our brain.
Hirers might think their gut instinct is a genuine skill, honed over time, but this feeling is more likely to be their biases kicking in.
Switch to structured interviews
Whilst you can’t interview blindfolded, there are measures you can take to make interviewing significantly more inclusive and impartial .
Your first step should be to ‘structure’ your interviews.
Structured interviews entail asking all candidates the same questions in the same order.
All candidates should have a similar interview experience, with as few tangents as possible.
Often, gender bias in interviews crops up when interviews aren't uniform.
Interviewers may phrase questions differently depending on the gender of the candidate.
An investigation into why female entrepreneurs continue to receive less funding than their male counterparts found that venture capitalists posed different types of questions to male and female entrepreneurs.
They tended to ask men questions about the potential for gains and women about the potential for losses.
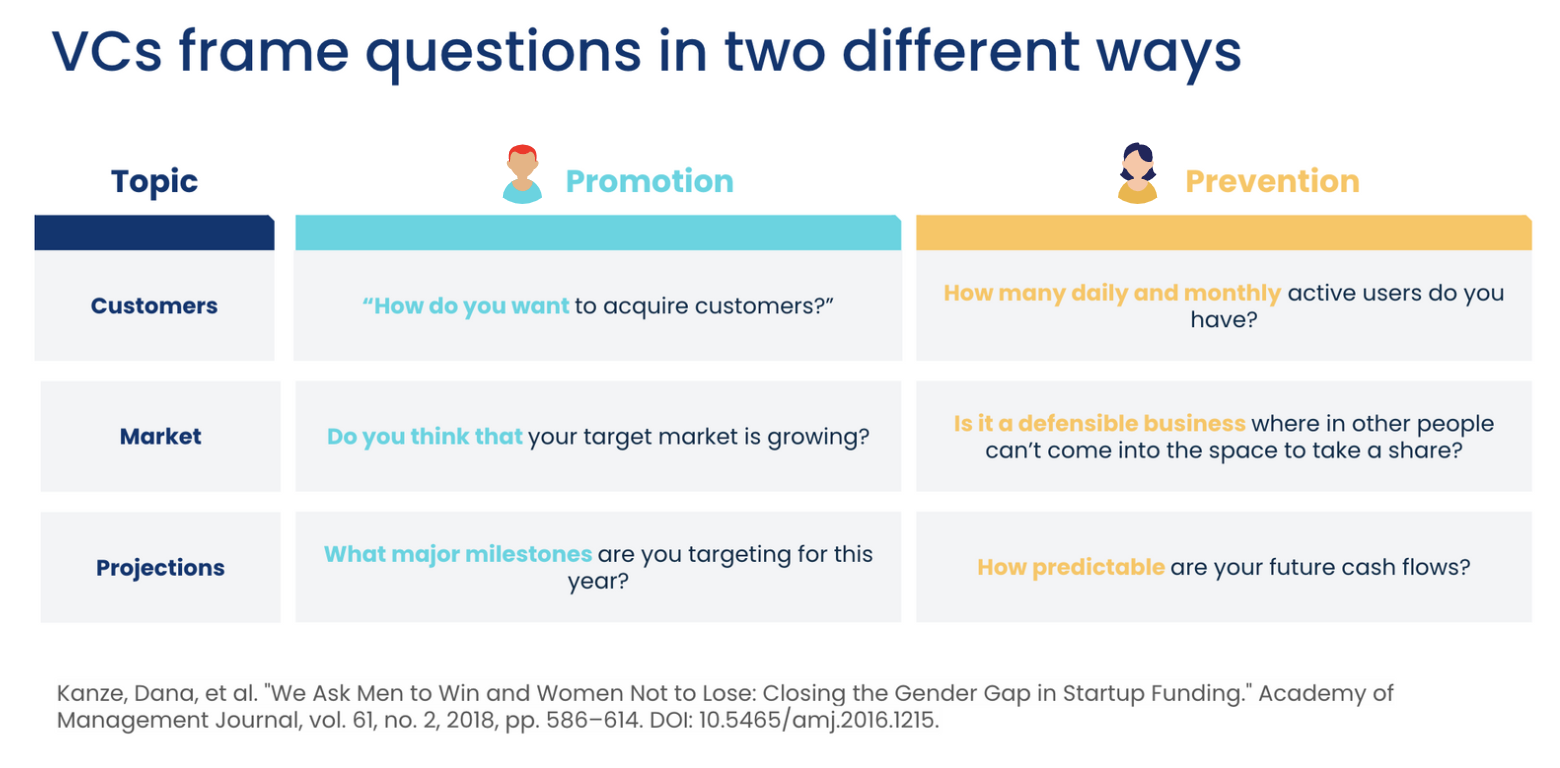
Making interviews more uniform not only makes it easier to objectively compare candidates but also ensures that every candidate gets the same opportunity to showcase their skills.
Once we decide we like someone, we'll look for reasons to keep liking them, and a single good or bad answer can taint our view of them.
Key takeaway: Make interviews as uniform as possible to compare like to like
Ask work sample-style questions
Much like the details on a CV, traditional interview questions that probe into a candidate’s background and experience will trigger bias.
Instead, use work sample questions or case studies to further test skills.
The benefit of using these at the interview stage is that you get to see not just the answers candidates give, but how they approach the task and arrive at those answers.

The task above could just have easily been ‘tell me a time when…’ questions.
However, these backwards-looking questions require candidates to exaggerate their past achievements and in many cases, completely lie.
By asking how they would approach a given scenario, irrelevant factors such as confidence and one’s ability to embellish their past experience are removed from the equation.
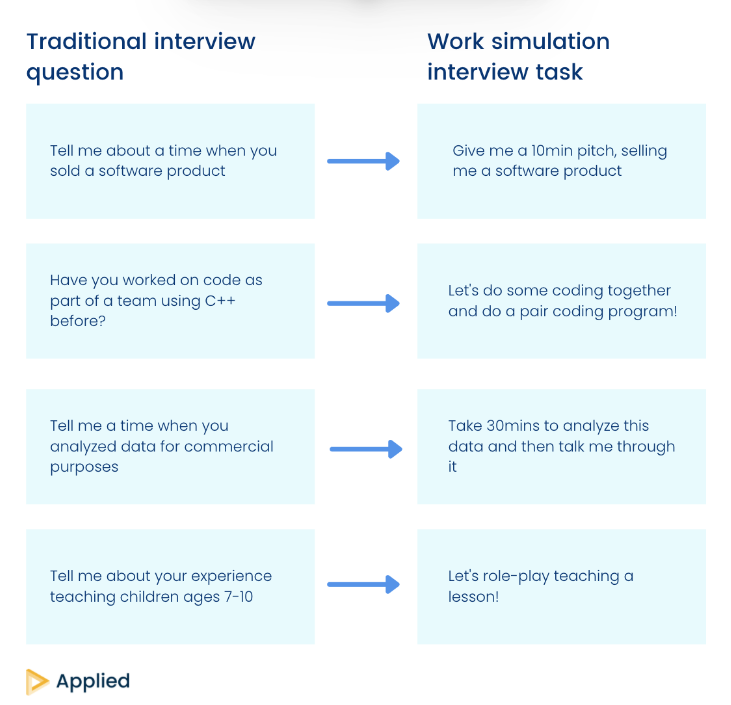
Past the initial screening, gender bias in interviews is often due to how candidates ‘come across.’
We know from the Heidi Roizen case study that even when identical, a male and female’s past achievements and personality will be perceived differently based on gender alone.
Which is why we use forward-looking, hypothetical questions - to test skills over personality and accomplishments.
Key takeaway: Ask forward-looking questions to mitigate biases around personality/ experience
Have multiple interviewers and scoring criteria
Using data is the most effective way to negate gut instinct (bias) from hiring.
To use data to make decisions, you’ll first have to make sure you’re collecting it over the course of the process.
Every work sample and interview question should have scoring criteria - a 1-5 star scale that details what a good, bad and average answer would look like.
This will not only give you data to help make decisions, but will also reduce bias, since hirers will have a set benchmark against which to judge candidates.
Here’s an example work sample with scoring criteria:
Question:
We're in the process of reaching out to management consulting firms as part of this week's marketing campaign:
Draft an email to the Head of HR, introducing Applied, and try to arrange an introductory call.
Scoring criteria:
# 5 Stars
- Concise message, with a clear structure and well written.
- Skilfully and sensitively personalised to their particular needs.
- Correct and clearly articulated understanding of what Applied is.
- Some use of evidence or 3rd party comparison to demonstrate benefits/generate interest.
- Not entirely focused on diversity benefits. Linking to the wider talent picture.
# 3 Stars
- Good understanding of what Applied is.
- Clearly written with good spelling/grammar and clarity/structure.
- Personalised to the prospect.
- Orientated around starting a conversation not "salesy"
- A clear call to action.
# 1 Star
- Not personalised to the business, or accusatory and insensitively handled
- Lack of clarity of what Applied is.
- Poorly written, in either spelling/grammar or clarity/structure.
- Overly “Salesy”. Trying to sell rather than initiate a relationship.
For the most unbiased scores have three team members score work samples, and then another three score each interview round.
‘Crowd Wisdom’ is the general rule that collective judgment is more accurate than that of an individual.
An interviewer may have unconscious biases, but by having multiple interviewers much of this bias will be averaged out.
The more diverse your reviewers panel, the less biased the scores.
If you’re specifically aiming to increase gender diversity, you should aim to assemble a gender diverse panel.
Note: three reviewers per round is recommended since adding any additional reviewers will bring diminishing returns.
This may sound challenging with a small team, but the panel doesn’t need to consist of entirely fresh team members each time, so long as the combination is changing.
You can also have a single team member join every panel - this would usually be the hiring manager who originally created the role.
Here’s how you can use crowd wisdom with a small team:
Work sample screening: Reviewer A, Reviewer B, Reviewer C
Interview round 1: Reviewer A, Reviewer B, Reviewer D
Interview round 2: Reviewer A, Reviewer C, Reviewer E
At the end of the process, each candidate’s scores can be added up to form a leaderboard.
Final hiring decisions can be made on these scores alone.
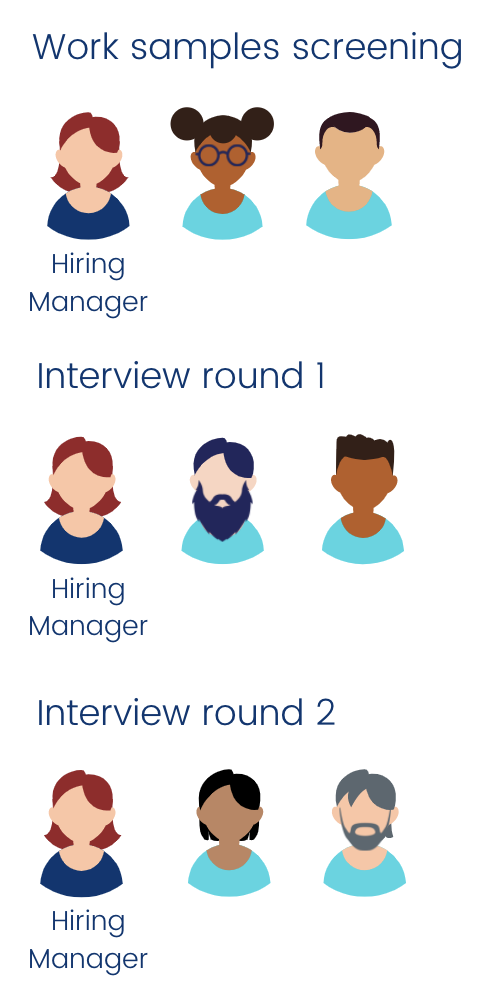
Key takeaway: Have three reviewers per assessment round for fairer, more accurate scores
Track gender diversity metrics
Tracking is essential to measuring how fair your process is.
If your goal is to attract and hire more women, you need to know how many women enter at the top of the funnel, and how they progress through the rest of the process.
Once you’re able to see how cohorts move through the various stages, you can identify any part of your process that may cause a disproportionate drop-off.
If you noticed that a woman scored particularly poorly on one particular work sample or interview question, you’d then be able to look at the question itself and ensure that it is inclusive.
It may be that the scoring criteria for that question favours a particular style of approach - corporate hiring processes in the U.S, for example, have been shown to prefer a masculine style of leadership.
Even at the very beginning of the process, data will help ensure gender equality.
You should look to source at least a 50/50 gender split. Studies have shown that when there’s just one woman in the finalist pool, their chances of being hired are statistically zero.
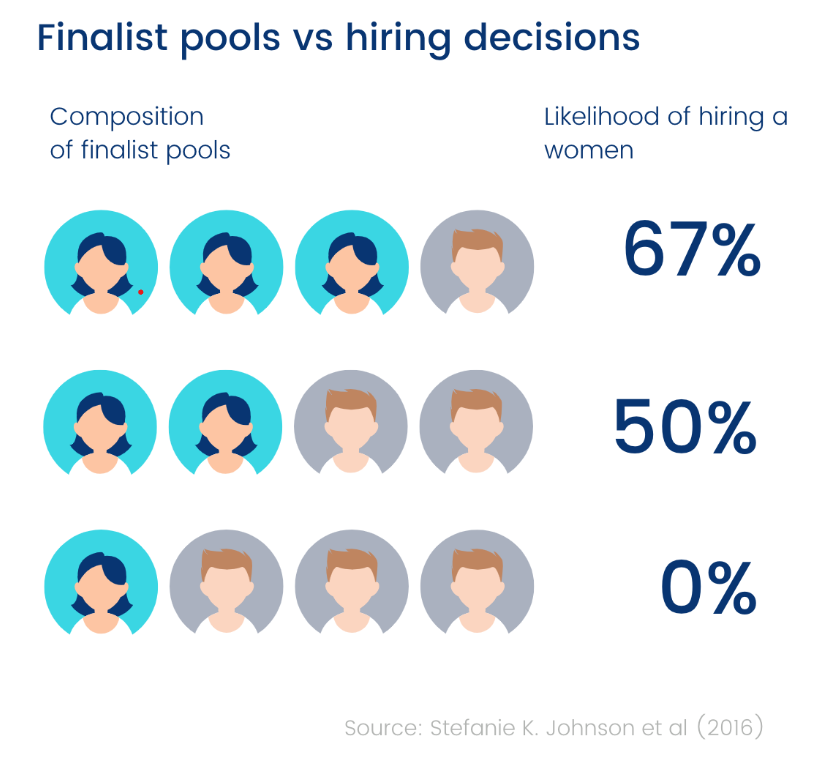
Why? Because it highlights how different she is from the norm.
Deviating from the norm is a risk for decision-makers, as people tend to alienate those who are different from the in-group.
So how should you go about collecting diversity data?
The easiest way to do this is by adding a basic equal opportunities form to your application process (we've shared ours below).
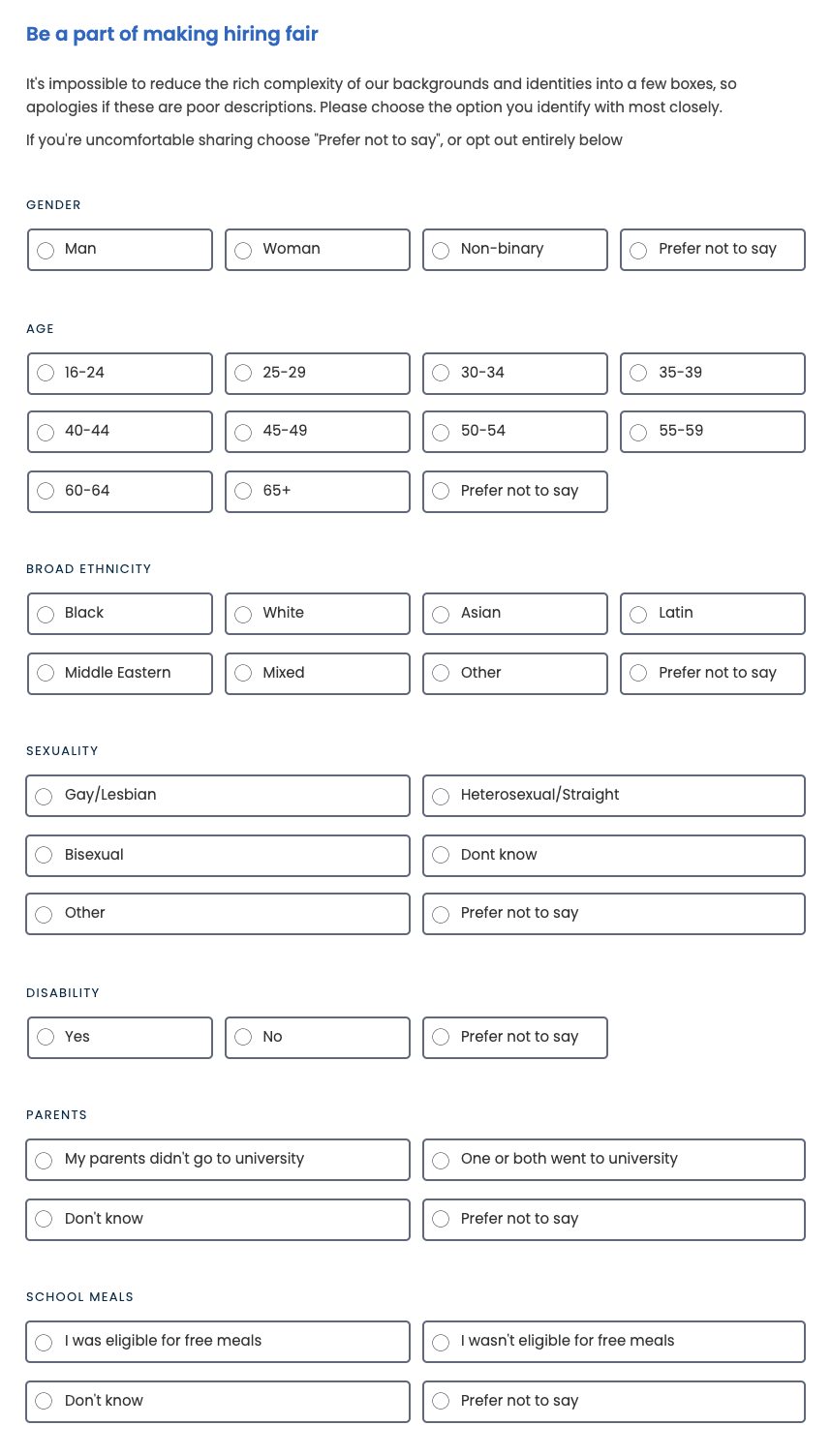
You can then track the relevant diversity metrics you're looking to improve.
If your primary concern is gender bias, you have to know how women actually perform in order to concentrate your efforts in the right places.
Candidates may be skeptical about sharing this information and it should never be mandatory.
Be sure to explain that their data is being collected to ensure the fairness of the process and that it will only ever be used at an aggregate level - so they will remain anonymous.
Key takeaway: you can’t fix what you can’t measure, so start tracking diversity metrics.
Why is gender equality important?
We know that gender equality is the right thing to do morally.
And this should be enough to inspire change.
Although making ‘the business case’ for diversity shouldn’t be necessary, the research still makes for interesting reading.
An Australian study claims to be the first to do real causal mapping that shows better gender diversity improves financial performance.
Whilst there’s no shortage of articles listing the benefits of gender diversity in the workplace (e.g. better reflection of customers, wider talent pool, improved collaboration), this research drives home the reality that gender equality is vital to a company’s success.
Researchers found that when there are women on Boards, companies are more likely to outperform their sectors.
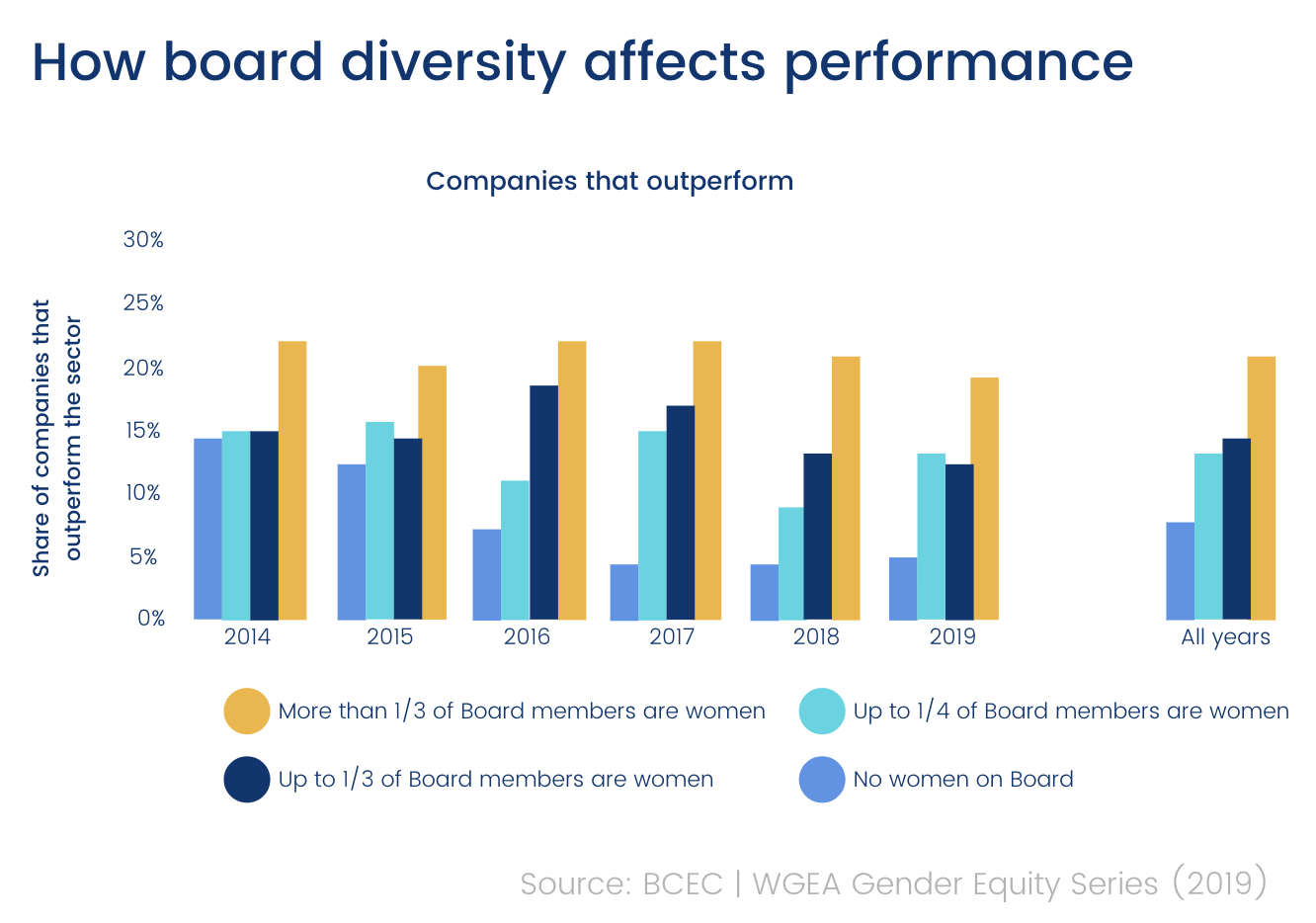
Companies that have no women on their Board are even three times more likely to be underperforming compared to those that have at least one-third female Boards.
Looking at management positions more broadly, McKinsey’s report found that organizations with more women in the C-Suite were more likely to experience above-average profitability and had a 27% likelihood of outperforming their peers on longer-term value creation.

A 2009 study even found that businesses with high levels of gender diversity are more likely to report higher than average percentages of market share and higher than average profitability.
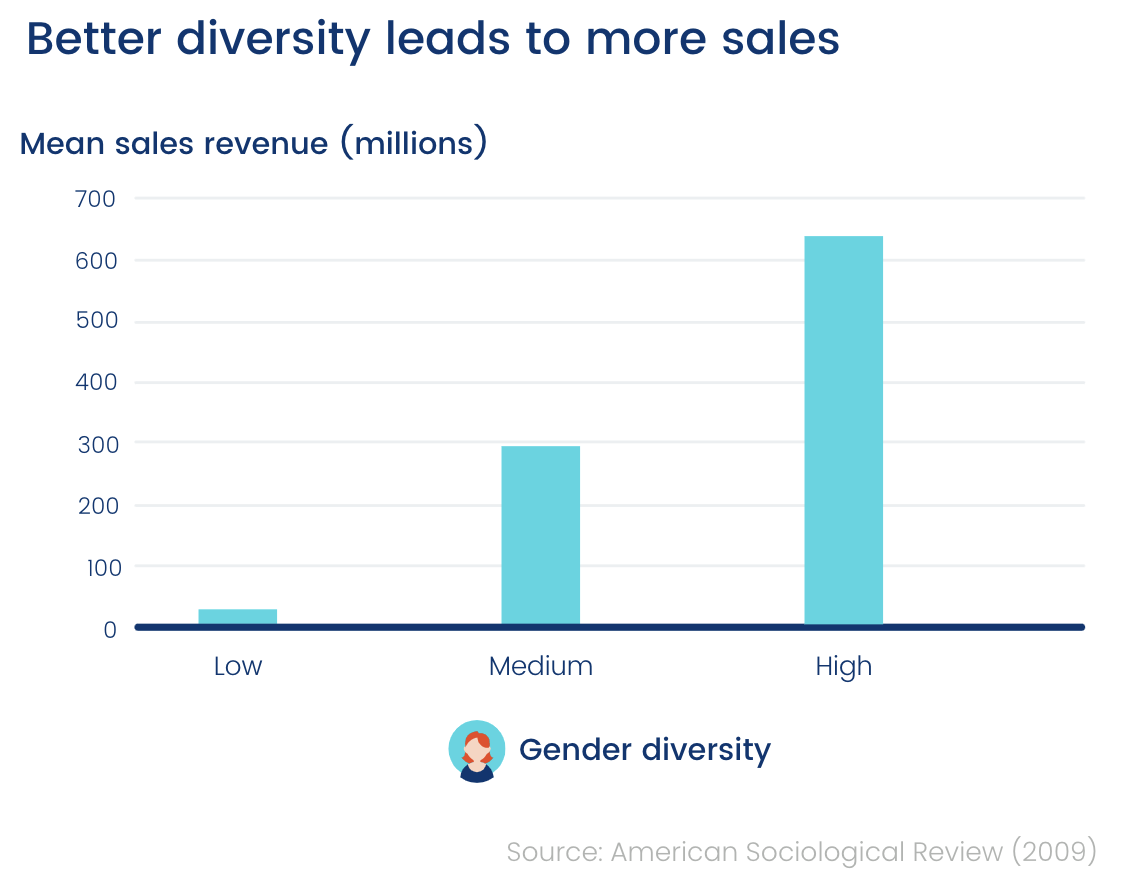
So gender bias in hiring isn’t just that harms the women who are unfairly disadvantaged...
When their gender diversity is poor, entire organizations lose out.
Applied is the essential platform for fairer hiring. Purpose-built to make hiring ethical and predictive, our platform uses anonymised applications and skills-based assessments to improve diversity and identify the best talent.
Start transforming your hiring now: book in a demo or browse our ready-to-use, science-backed talent assessments.