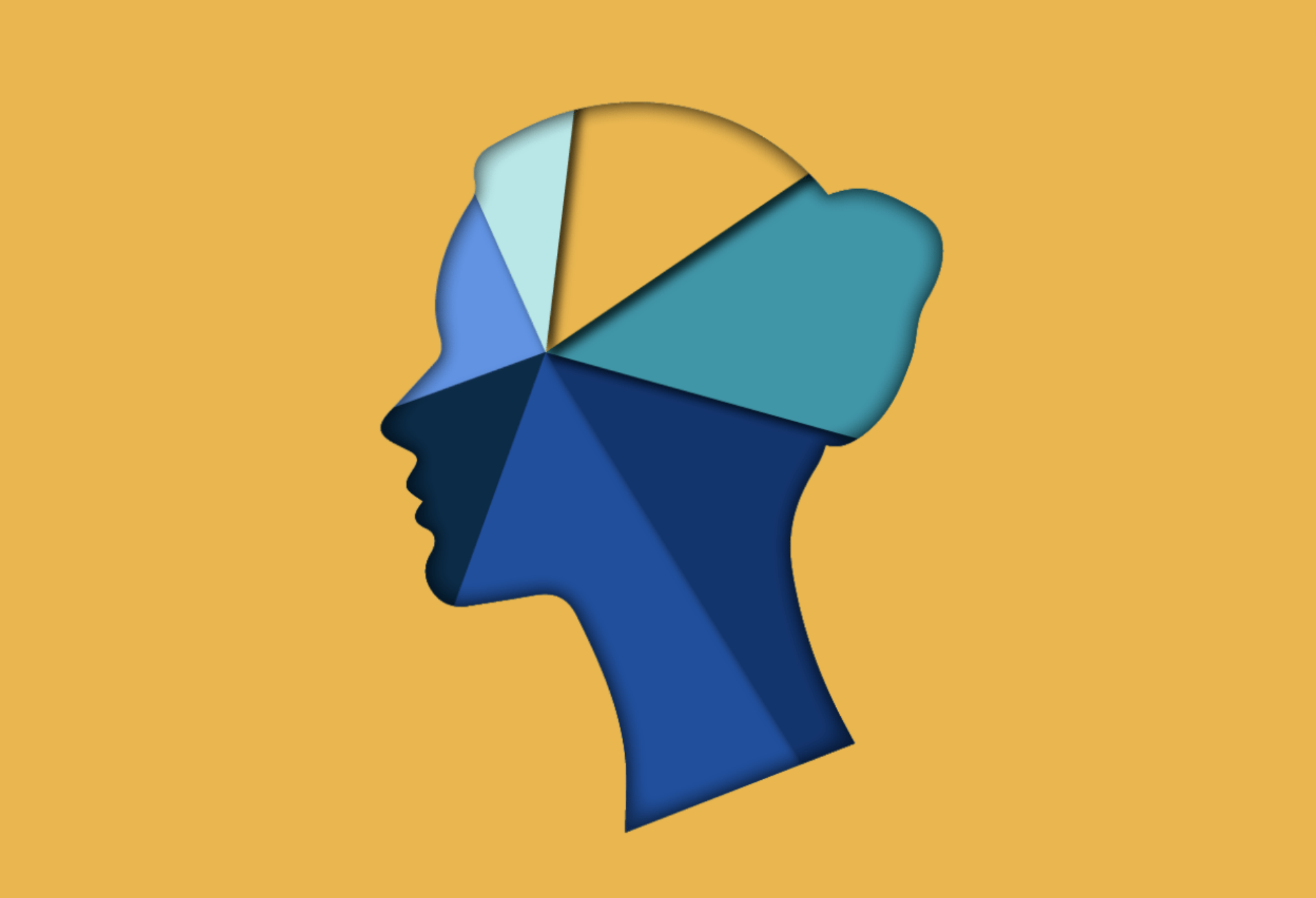
Traditional hiring is biased.
No matter how well-intentioned you may be, we’re all subject to unconscious biases that affect our decision-making.
Below, we’ll explore the key studies around recruitment bias and share research-backed strategies for removing this bias.
Here at Applied, we practice what we preach: all of the advice we’ll share below is based on behavioural science and is exactly what we do to ensure our hiring is fair, inclusive and predictive….
In this report:
- Racial bias in hiring (UK/USA data)
- Gender bias in hiring (UK/USA data)
- The impact of ordering effects
- The impact of AI
- How to remove bias from your hiring process
- 1) The danger of CVs
- 2) Fairer interviewing
- 3) A word on culture fit
The Impact of Racial Bias on Recruitment: Understanding the Consequences of Biased Hiring Decisions
UK
Although not the most recent, the biggest study of racial bias in hiring here in the UK is this 2009 report.
Applications were randomly assigned an ethnicity and sent off to a range of employers - controlling for everything except the candidates’ ethnicity.
Each ethnicity was tested against white candidates to mitigate any variation across different employers (this is why you can see varying success rates for white candidates in the chart below).

The result: white candidates were favoured in around 47% of the tests.
And Indian candidates would have to send twice as many applications to get the same callbacks as white-named applications.
- Equal treatment: 35%
- Ethnic minority favoured: 18%
- White favoured: 47%
Researchers from the London School of Economics took this study further - combining it with another field study - a part of a larger European project conducted in 2016/2017.
They concluded that ethnic minority talent still faced similar levels of discrimination in the hiring process and tend to rely more heavily on their social networks as a result.
The disparities in outcomes we see for specific ethnic groups are therefore likely to be a product of socioeconomic differences between these groups - something we can directly measure (see chart below).
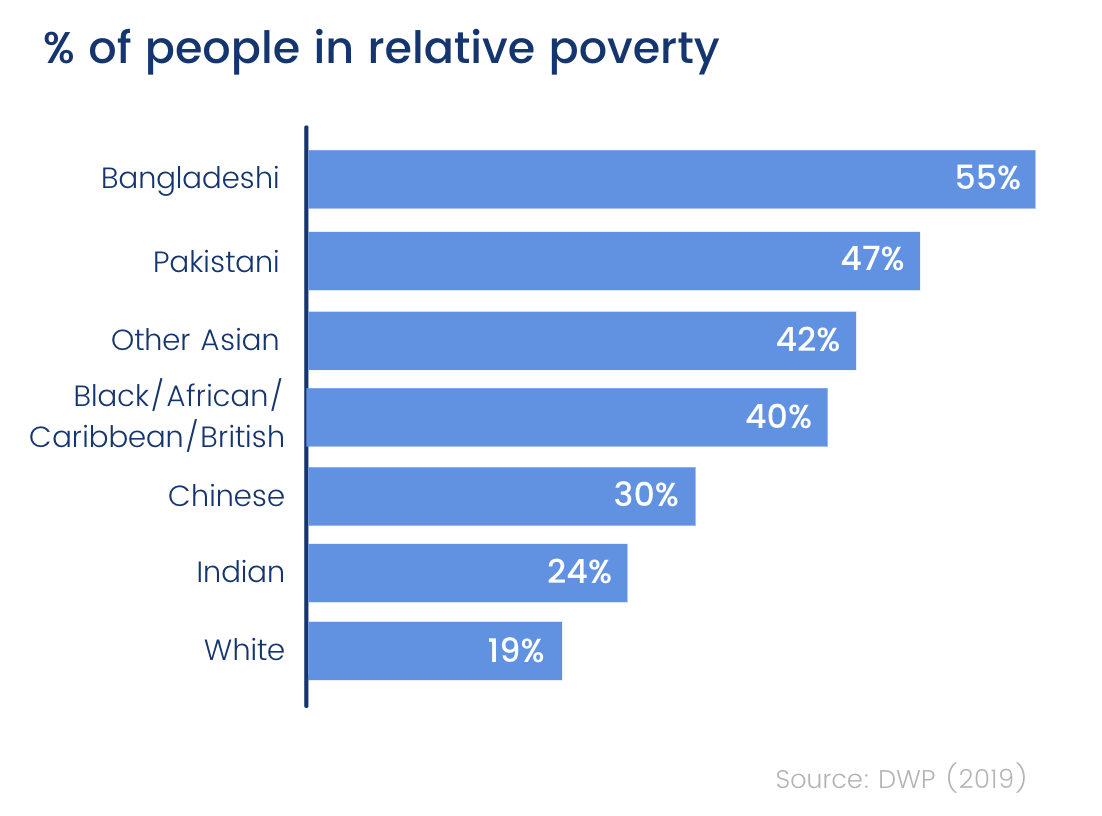
Another, more recent study from the University of Oxford had similar findings around recruitment bias…
After sending out identical applications with only the candidate’s ethnicity being changed, they found that candidates from minority ethnic backgrounds had to send 80% more applications to get the same results as a White-British person.
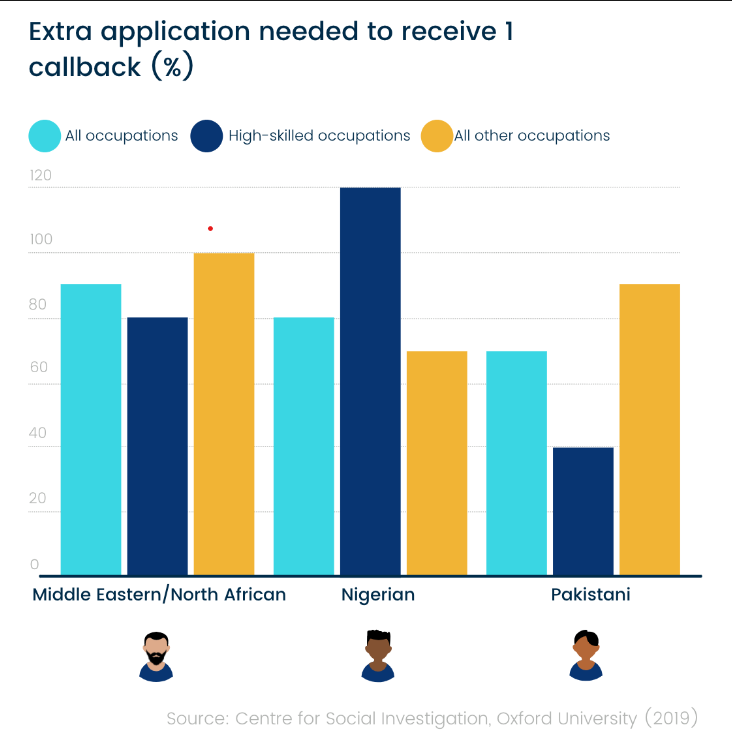
24% of applicants of white British origin received a positive response from employers…
Compared to just 15% of minority ethnic applicants.
And the researchers also found that this bias had barely improved from when they conducted similar experiments back in 1969.
Inside Out UK replicated these correspondence studies on a smaller scale, focussing solely on Muslim-sounding names (which although not technically a ‘race’, is an ethnicity).
Below are the results…
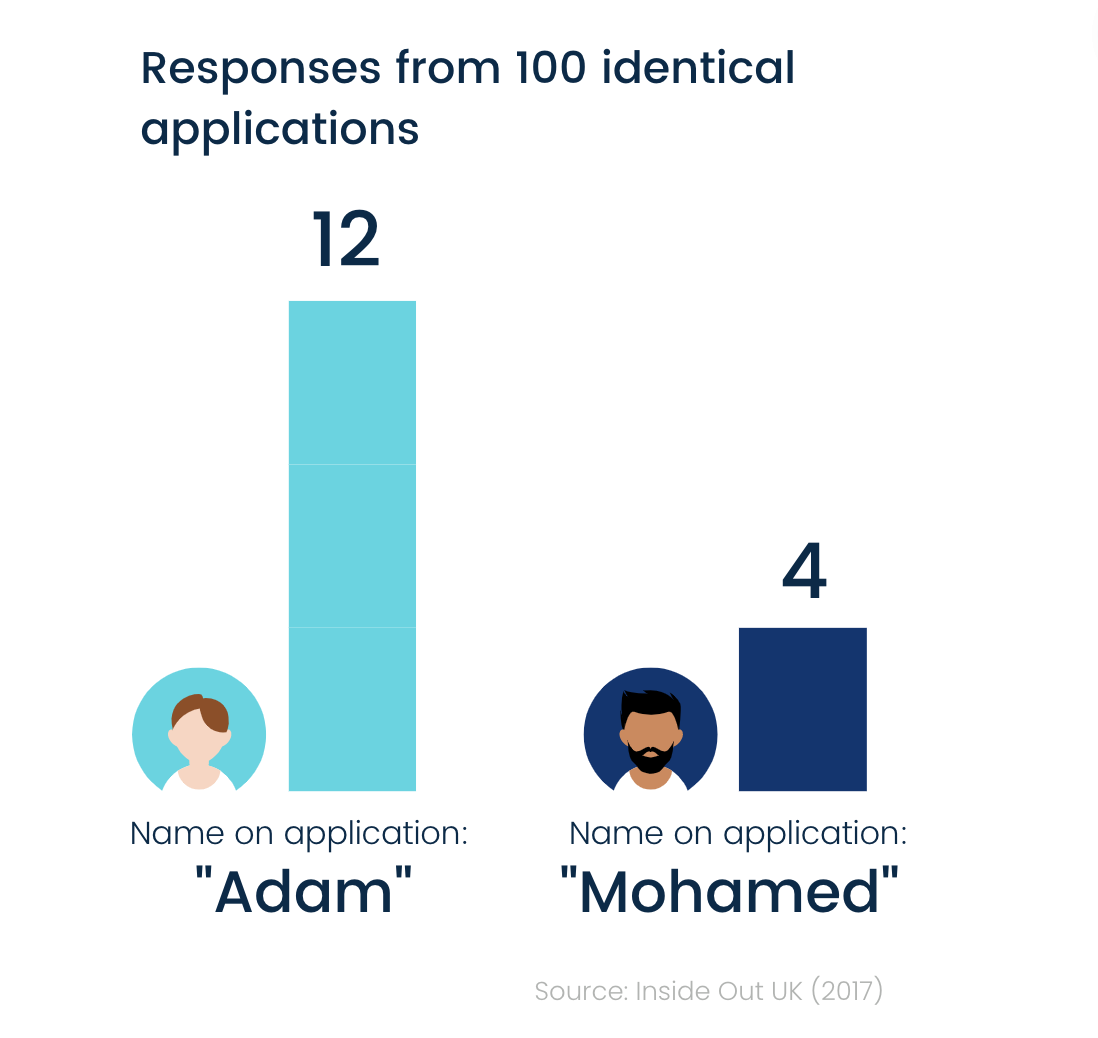
100 job opportunities were applied for using identical CVs (the name being the only variable that changed)
- Adam: offered 12 interviews
- Mohamed: offered 4 interviews
This means that candidates with a Muslim-sounding name are 3x more likely to be overlooked for a job.
USA
A 2011 study conducted in Canada sent off thousands of randomly manipulated CVs in response to online job postings.
Researchers found significant discrimination across a variety of occupations towards applicants with foreign experience or those with foreign names.
(We’ve simplified the findings in the chart below, but you can see the full results here)
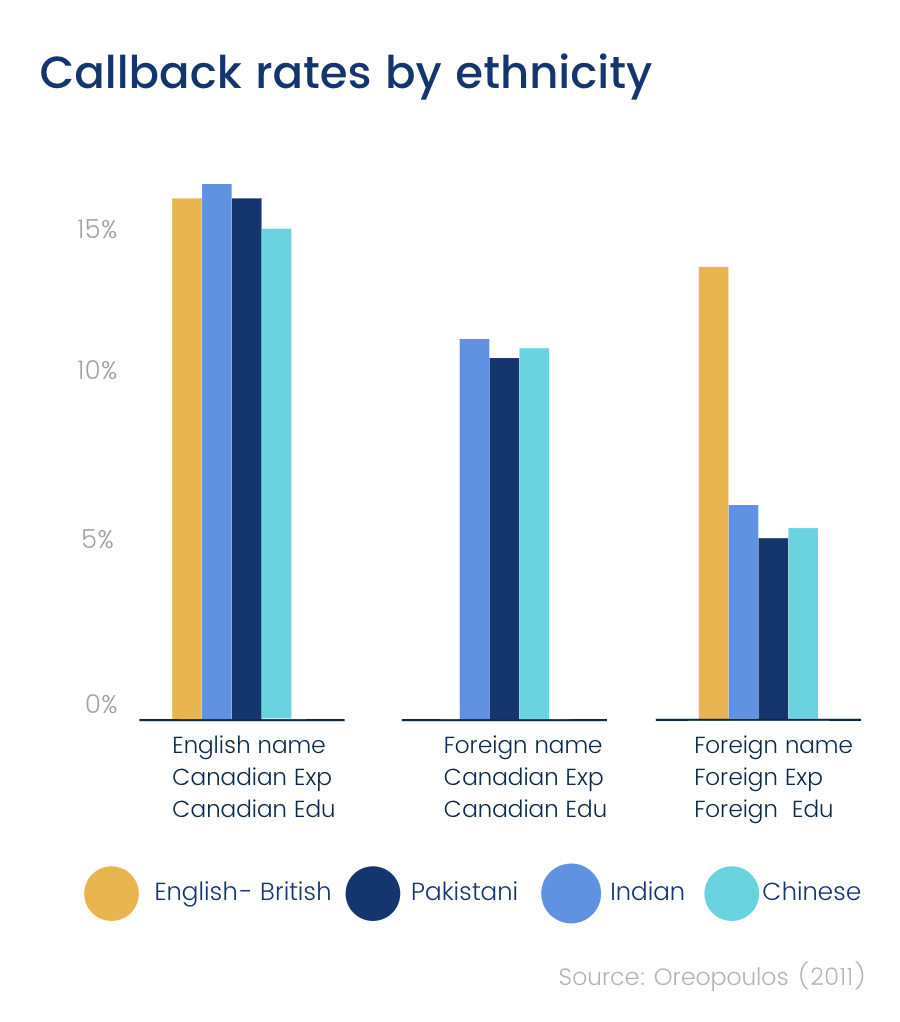
The rough baseline callback rate for an all-Candian/English CV was 16%.
Changing only the name to one with Indian origin lowered the callback rate by 4.5% (to 11.5%).
Changing it to one with Pakistani or Chinese origin lowered this slightly more, to 11.0% and 11.3% respectively.
Overall, CVs with English-sounding names are 39% more likely to receive a callback.
The same went for education and experience. The more ‘foreign someone’s background, the lower their chances of getting a callback.
Looking specifically at the effect of Black-sounding names on callbacks, one study involving over 5000 CVs found that applicants with white-sounding names needed to send roughly 10 CVs to get a callback, whilst applicants with African-American names needed to send about 15.

In a 2021 study, over 80,000 fake job applications were submitted for entry-level positions to 100 different companies.
The study found that generally, candidates with black-or African-American-sounding names received fewer callbacks compared to those with white-sounding names.
White applicants experienced a 9% increase in responses when compared to their peers.
What’s interesting about this study is that it delved into which companies were actually doing the discriminating.
Bias was heavily concentrated in a small number of (generally larger) employers. Around half of the total discrimination against Black candidates could be attributed to 20% of organisations.
A study from 2016 tested whether or not employers with pro-diversity language in their job ads showed any less recruitment bias.
Researchers found that when applying to an organisation that presents itself as valuing diversity, minority background candidates were less likely to ‘whiten’ their CV.
However, these organisations’ diversity statements were not actually associated with reduced discrimination.
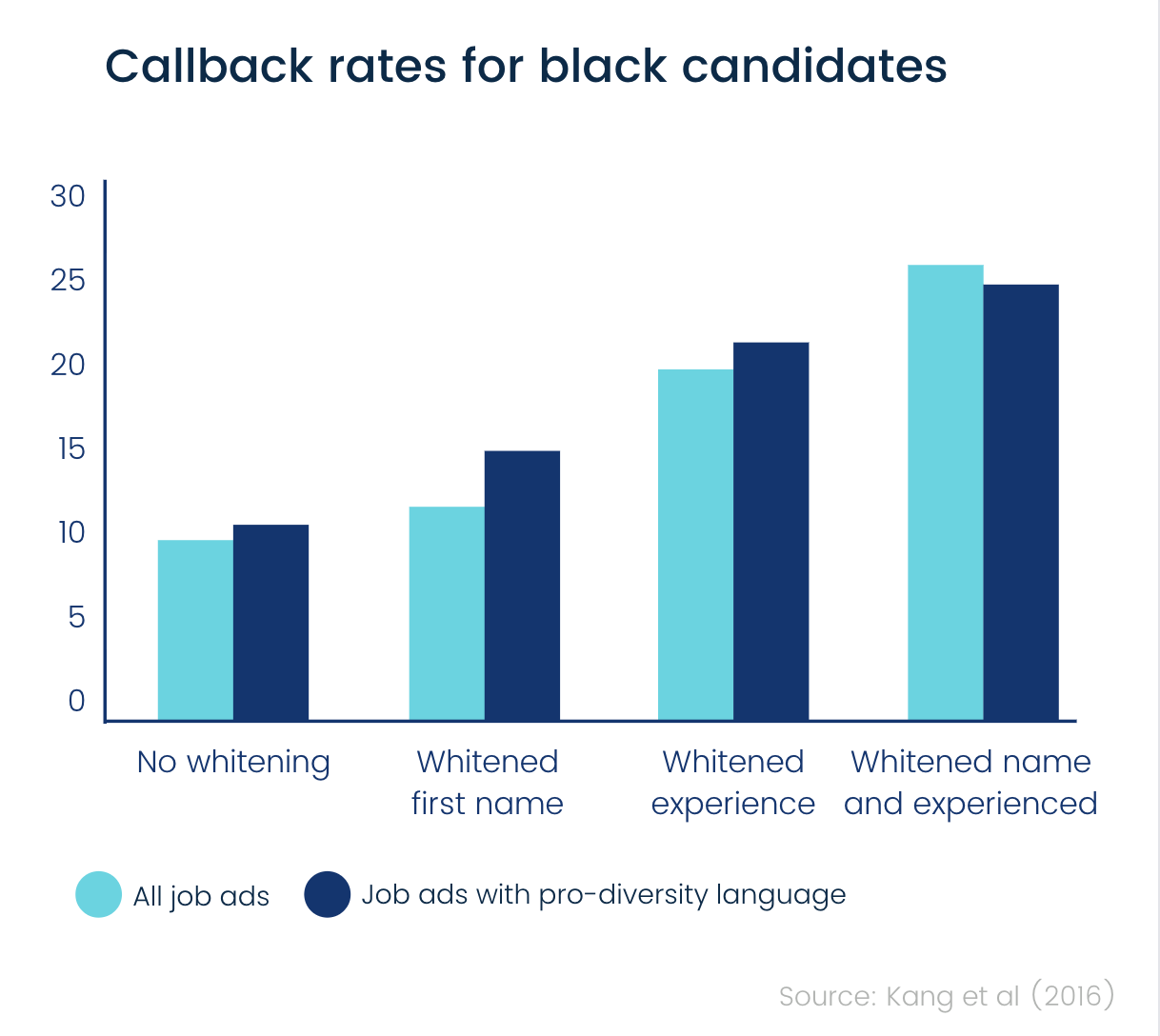
For more studies like these, check out our Racial Discrimination in the Workplace Report
Gender Bias in Recruiting: Overcoming Hiring Biases to Promote Diversity and Inclusion
UK
At a glance, it may seem as gender bias is a thing of the past.
Looking at the results UK study, you can see that at a very high level, men are only slightly ahead of women when it comes to callback rates.
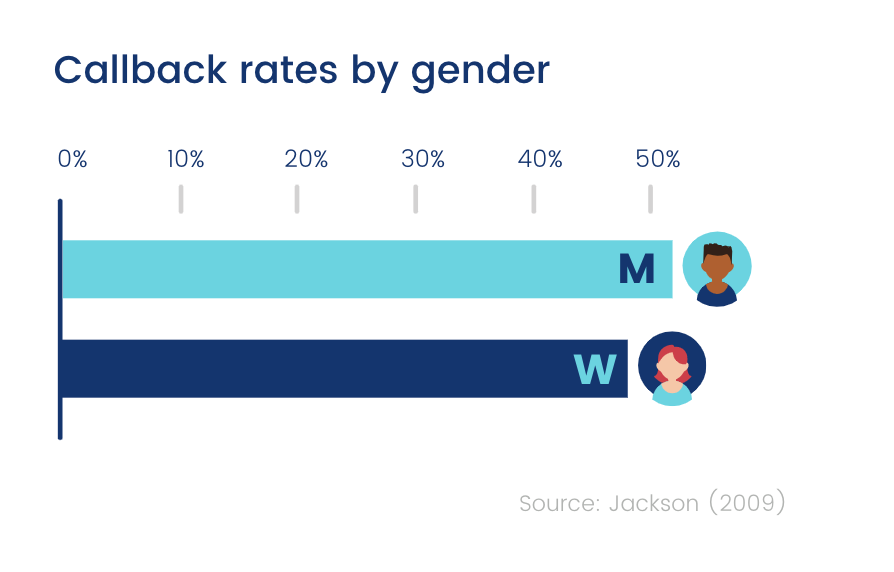
However, if we then look at individual roles, it becomes apparent that there is in fact a measurable bias against women.
Below are the results of a study carried out across the English labour market
Identical applications were sent to a wide variety of roles, changing only the sex on the application.
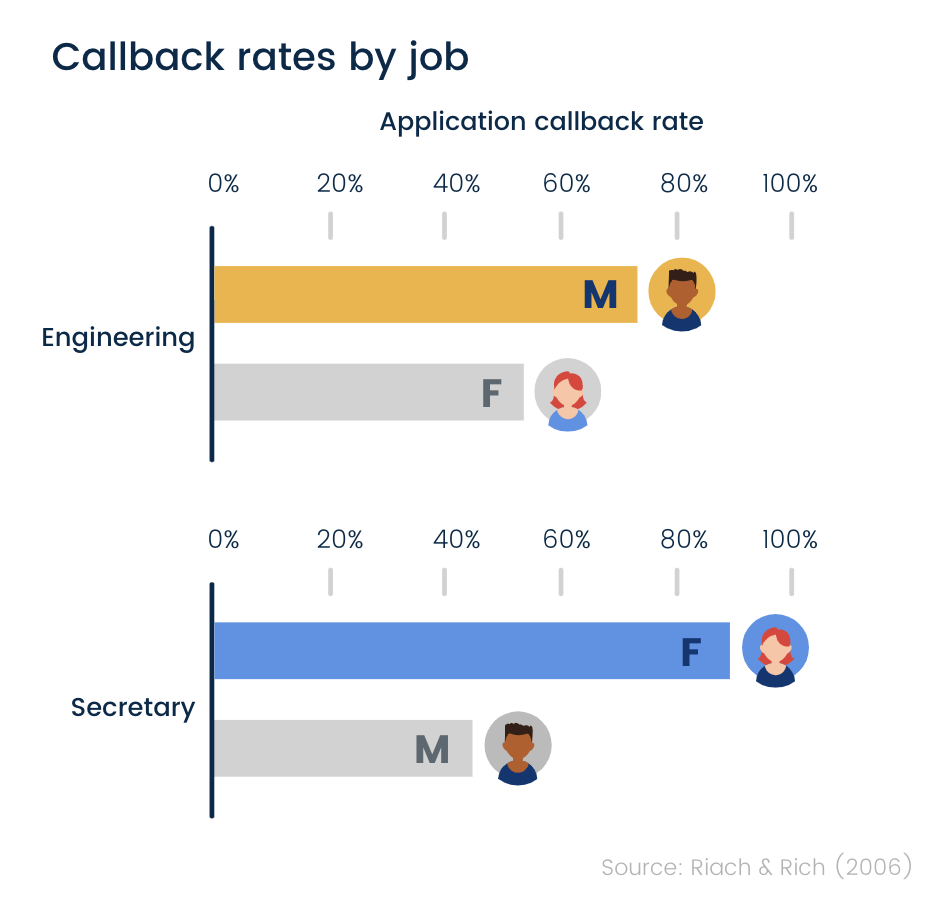
For ‘typically female’ roles (like a secretary in this case), women are favoured.
And for ‘typically male’ roles, men are favoured.
So gender bias seems to occur when people attempt to step out of the roles they’re stereotypically associated with.
We also know that, according to Mercer’s 2020 report, the higher up the corporate ladder you go, the fewer women you’ll come across.
If women are not who we expected to see in more senior roles, then it’s safe to assume that they’ll be subject to recruitment bias.
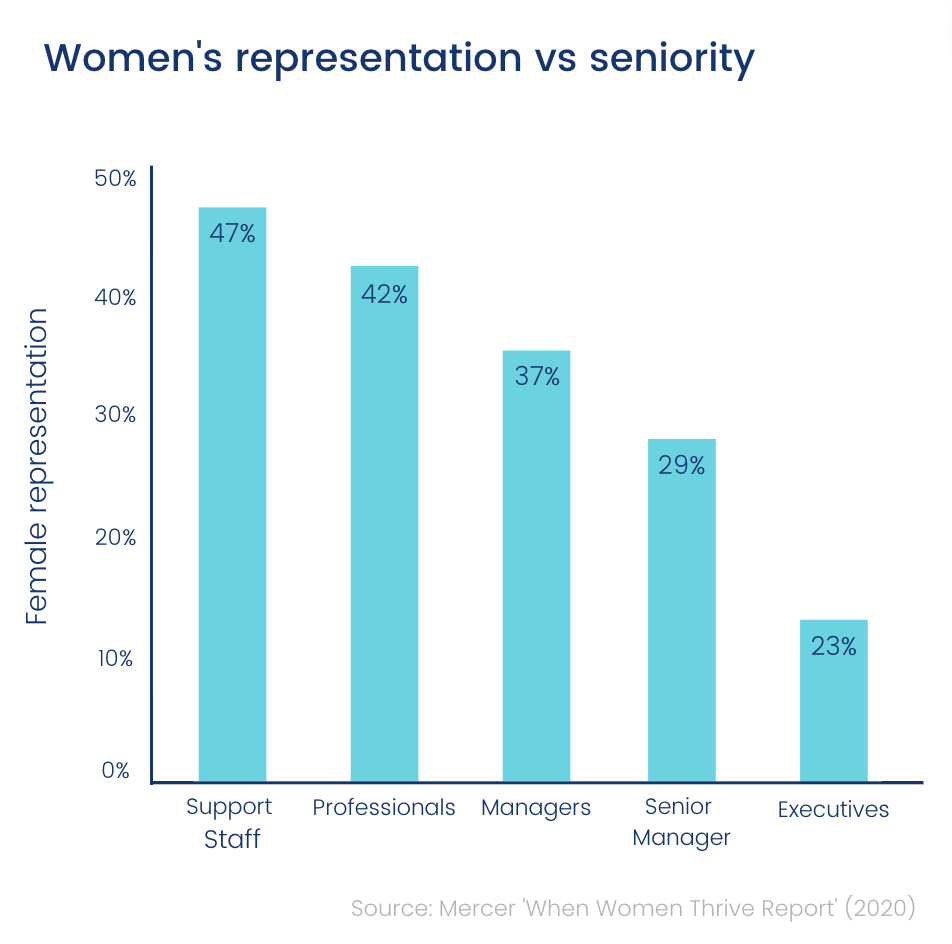
USA
The most famous US study on gender bias in hiring looked at science faculties at universities.
These science faculties were asked to rate candidates' applications to a laboratory manager position - the applications were randomly assigned a sex.
They were rated for competence, hireability, and the likelihood of receiving mentorship.
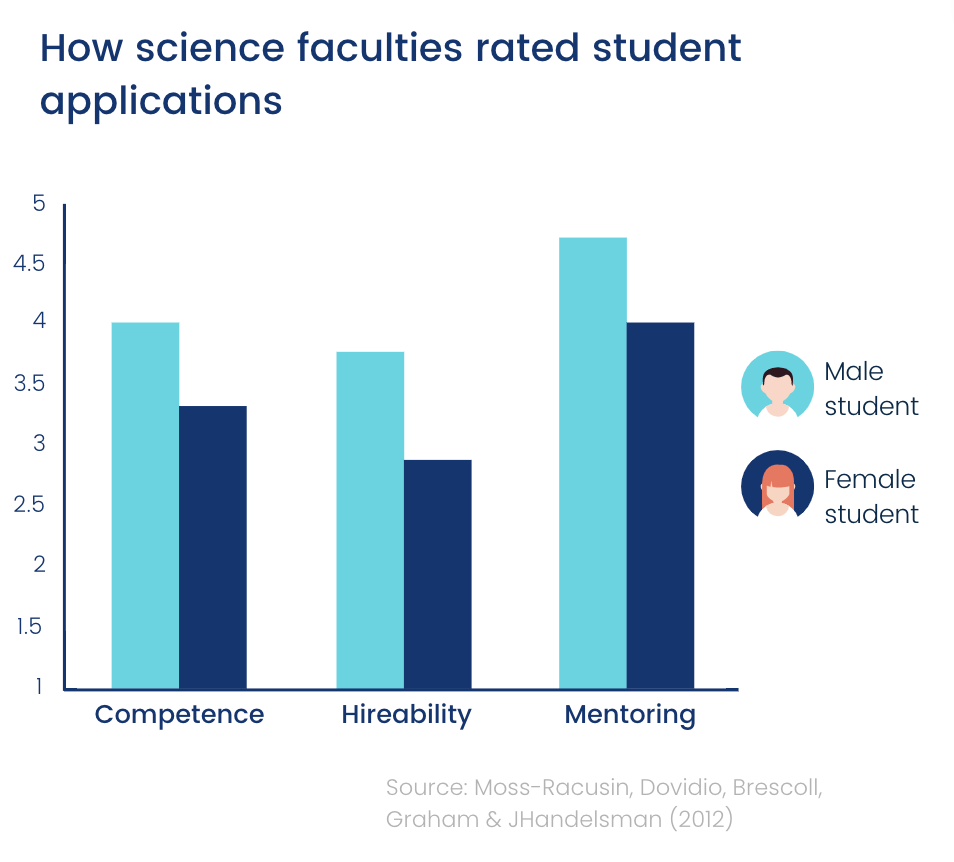
Despite the applications being identical (bar sex), women were rated as being less competent and hireable than male candidates.
Men were even offered higher starting salaries.
It’s also interesting to note that men and women were equally likely to show recruitment bias against female candidates.
This seems to point to much of this bias being unconscious, rather than an explicit plot to keep women out of science faculty jobs.
Another study delved into recruitment bias in law firms.
Researchers created two fictitious candidates, completely identical apart from their sex and class indicators.
They found that only men perceived as higher class benefitted from this background.
And for women, a higher-class background actually led to a “commitment penalty.”
After interviewing the employers, the researchers discovered that higher-class women were seen as being less committed to working and more likely to leave after having children.

For a more comprehensive look at the research, you can read our Gender Bias in Hiring Report.
Ordering Effects in Recruitment: How the Order of Job Candidates Influences Hiring Decisions and Contributes to Biases
Whilst the evidence clearly shows that certain demographics are disproportionately disadvantaged by traditional hiring processes, there are also ordering effects at play that can affect any candidate.
Even if we were to anonymise applications (something we’ll get onto soon), the context in which we’re presented with information can still influence our decision-making.
Just a quick look at our own data tells us that candidates reviewed first tend to receive better scores.
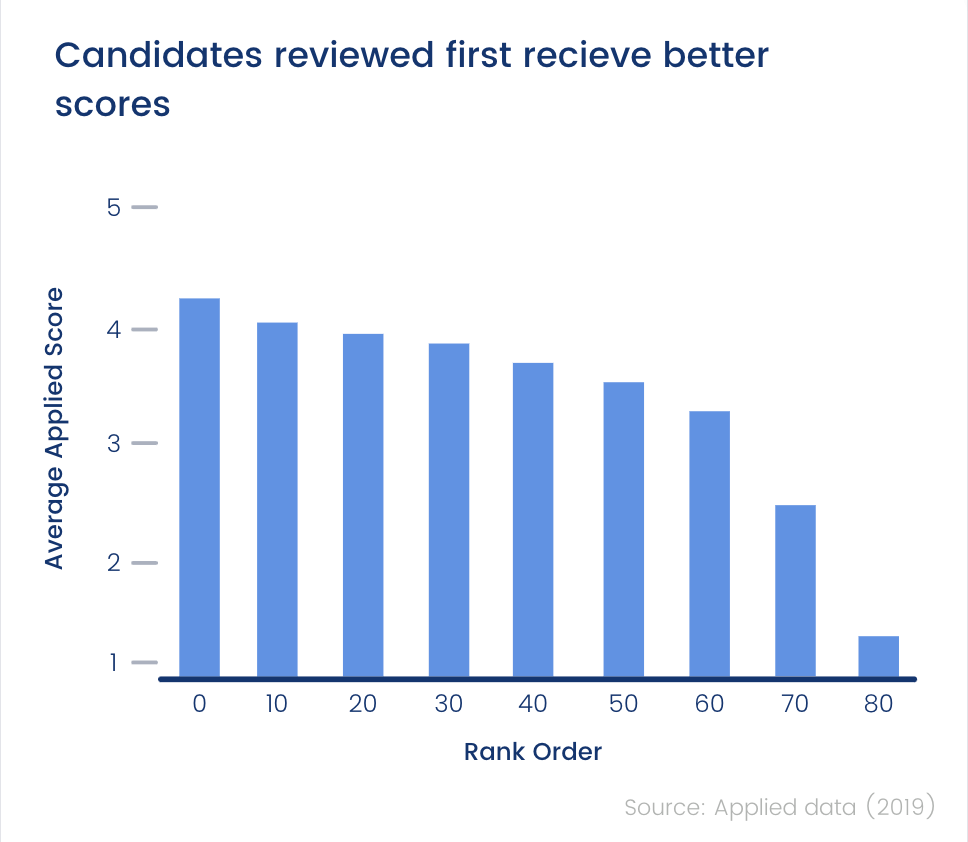
This is why we randomise the order in which they're reviewed in.
One of the most compelling studies on ordering effects is this study of Israeli judges.
Judges parole-granting decisions were analysed over the course of a day.
Below are the results…
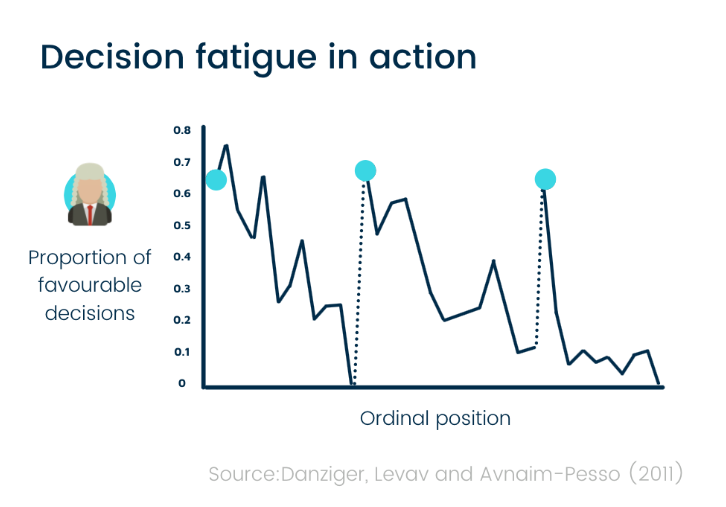
What exactly are we looking at here? Well, we can see that their decisions became harsher over time.
The more tired we become, the more risk-averse we get - a phenomenon known as ‘decision fatigue.’
The spikes we can see in favourable decisions were following the judges’ breaks.
It’s not hard to imagine how this could be applied to recruitment bias.
A tired hirer with a pile of CVs to get through will likely grow harsher as time goes on.
We’re also more likely to remember intense moments and the end of moments of an experience more vividly.
The ‘peaks’ and the end weigh higher in our mental calculations.
These snapshots dominate the actual value of an experience.
This unconscious bias is known as the ‘peak-end effect’.
One study put this to the test - asking participants to take part in two trials which involved putting their hand into uncomfortably cold water.
Trial 1: Place hand in 14° water for 60 seconds.
Trial 2: Place hand in 14° water for 60 seconds and then 15° water for 30 seconds
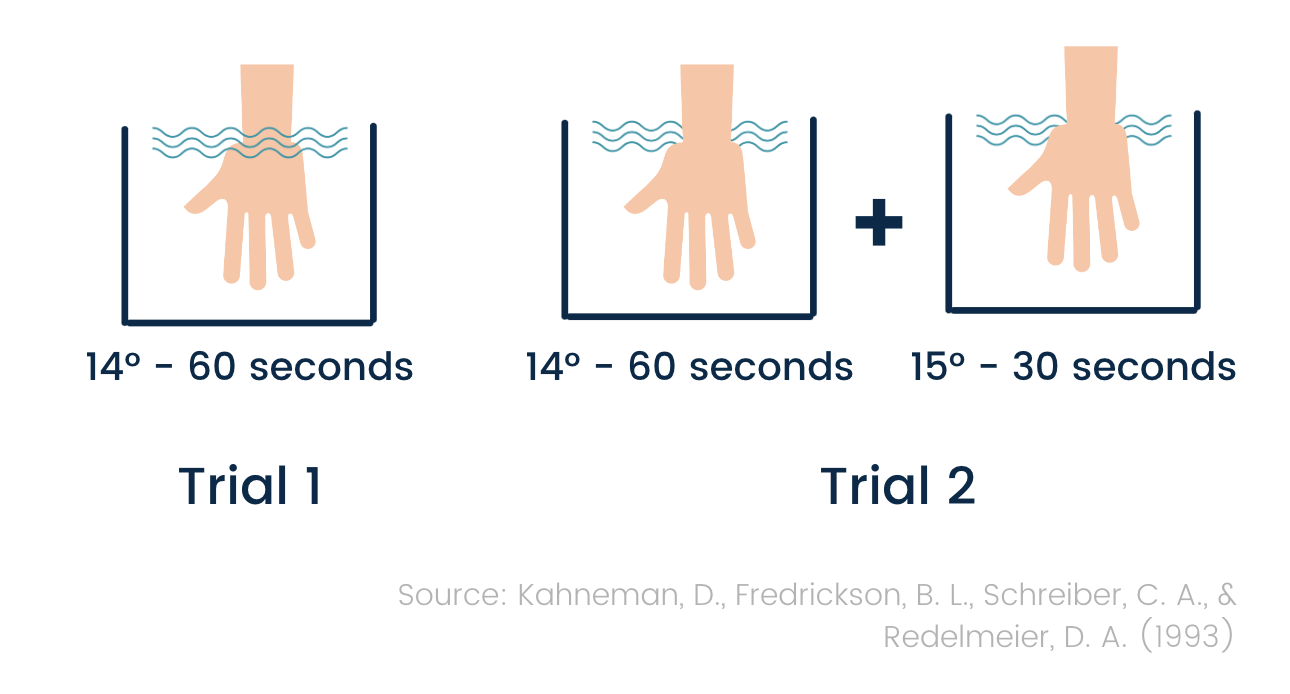
Participants reported that despite being uncomfortable for longer, they found Trial 2 less painful.
Why? Because the end of the experience was less painful (and the peaks were the same)
Coming back to recruitment bias, peaks and end will be spread across multiple candidates, causing an unfair memory of each applicant individually.
Those whose interviews had high points or ended well may be viewed more favourably as a result - despite not necessarily being more suitable.
The Role of AI in Mitigating Hiring Bias: The Mixed Evidence and Potential Benefits of Automated Recruitment
Talk of AI in recruitment is becoming more mainstream over time, with some advocates claiming that it can reduce bias.
This makes sense on the surface - you’d think that non-human hiring decisions would be more objective and less biased.
AI doesn’t have an ethnic identity, gender or even consciousness - and so must therefore be free of the unconscious biases that plague human decision-making.
However, this isn’t what we see play out in the real world.
In fact, according to a 2019 study by Harvard Business Review, these algorithms tend to reflect human biases, not mitigate them.
In 2018, for example, Amazon scrapped their development of an AI-powered recruitment process when it was found to discriminate against female applicants.
Why does this happen? Well, the issue often lies in the process of using ‘training data’ to build recommendations.
We have to provide our AI with a dataset it can learn from.
The problem is, if this dataset is based on human decision-making, chances are its going to biased... and thats how we end up with discriminatory algorithms.
The kinds of attributes we think are associated with being for being hireable are inherently bound up with gender and race.
In the case of Amazon, hires were found by looking at the backgrounds of candidates who submitted resumes in the last decade, a group that heavily skewed male.
So if we want to reduce recruitment bias and improve representation, its probably best - at least for now - to steer clear. of AI.
Strategies for Eliminating Recruitment Bias: Proven Methods for Achieving Fair and Impartial Hiring Practices
We’re all biased, so we have to design processes with this is mind
Unconscious bias affects everyone.
It doesn’t matter how fair or objective you believe yourself to be - unconscious bias is simply a part of being human.
When we look at the sort of outcomes the studies above result in, there are likely a number of biases causing this.
Perception bias: we believe something is typical of a particular group of people based on cultural stereotypes or assumptions.
Affinity bias: we feel as though we have a natural connection with people who are similar to us.
Halo effect: we project positive qualities onto people without actually knowing them.
Confirmation bias: we look to confirm our own opinions and pre-existing ideas about a particular group of people.
Attribution bias: we often attribute others’ behaviour to internal characteristics and our own behaviour to our environment - we are more forgiving of ourselves than others.
For the most part, we don’t know we’re falling prey to one of these biases (hence ‘unconscious’).
Unconscious bias tends to occur when we use the fast, shortcut-based part of the brain to make decisions that should be made using the slower, more conscious part.
This unconscious defaulting happens without us being aware of it.
What some hirers may refer to as their ‘gut instinct’ is essentially just unconscious bias - it’s using quick-fire associations and mental shortcuts to make snap judgments.
A candidate went to Oxford University? That means they must be intelligent.
This bias doesn’t make us bad people but left unchecked, it leads to diversity gaps widening and poor hiring decisions.
Unconscious bias training doesn't work
If we look at what some of the big tech companies in the U.S are doing, we can see that training is top of the agenda.
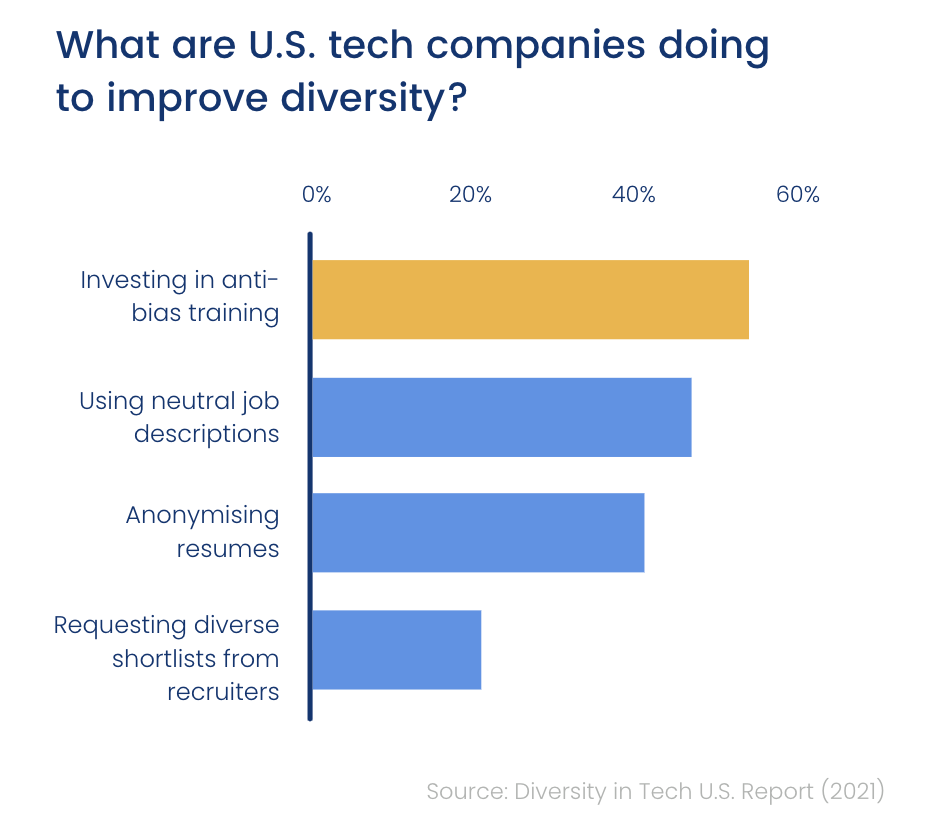
However, due to the unconscious nature of bias, training alone doesn’t work.If we look at one of the biggest studies on behaviour change (the chart above), you can see that the companies focused on diversity training actually saw less diversity in terms of black women in management positions.
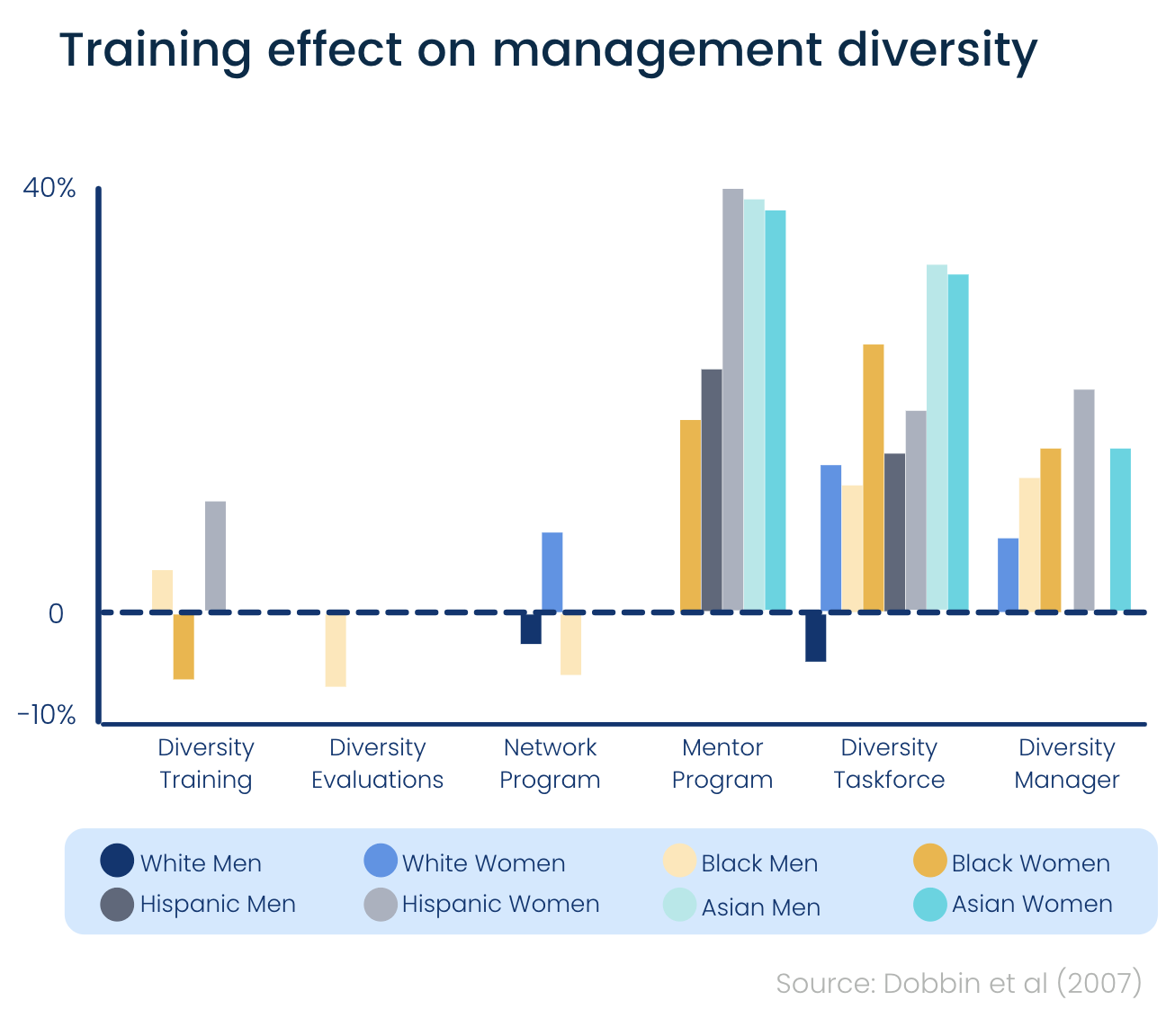
If we look at one of the biggest studies on behaviour change (the chart above), you can see that the companies focused on diversity training actually saw less diversity in terms of black women in management positions.
Humans are biased, and no amount of training will change that.
What does work, however, is carefully designed processes.
You can’t de-bias a person but you can de-bias the process they use to make decisions.
And this is exactly how we reduce recruitment bias here at Applied.
It might be time to rethink how you review CVs
If the research covered in this report has taught us anything, it’s that CVs are far from a perfect means of identifying talent.
The more we know about a candidate, the more grounds for bias we have.
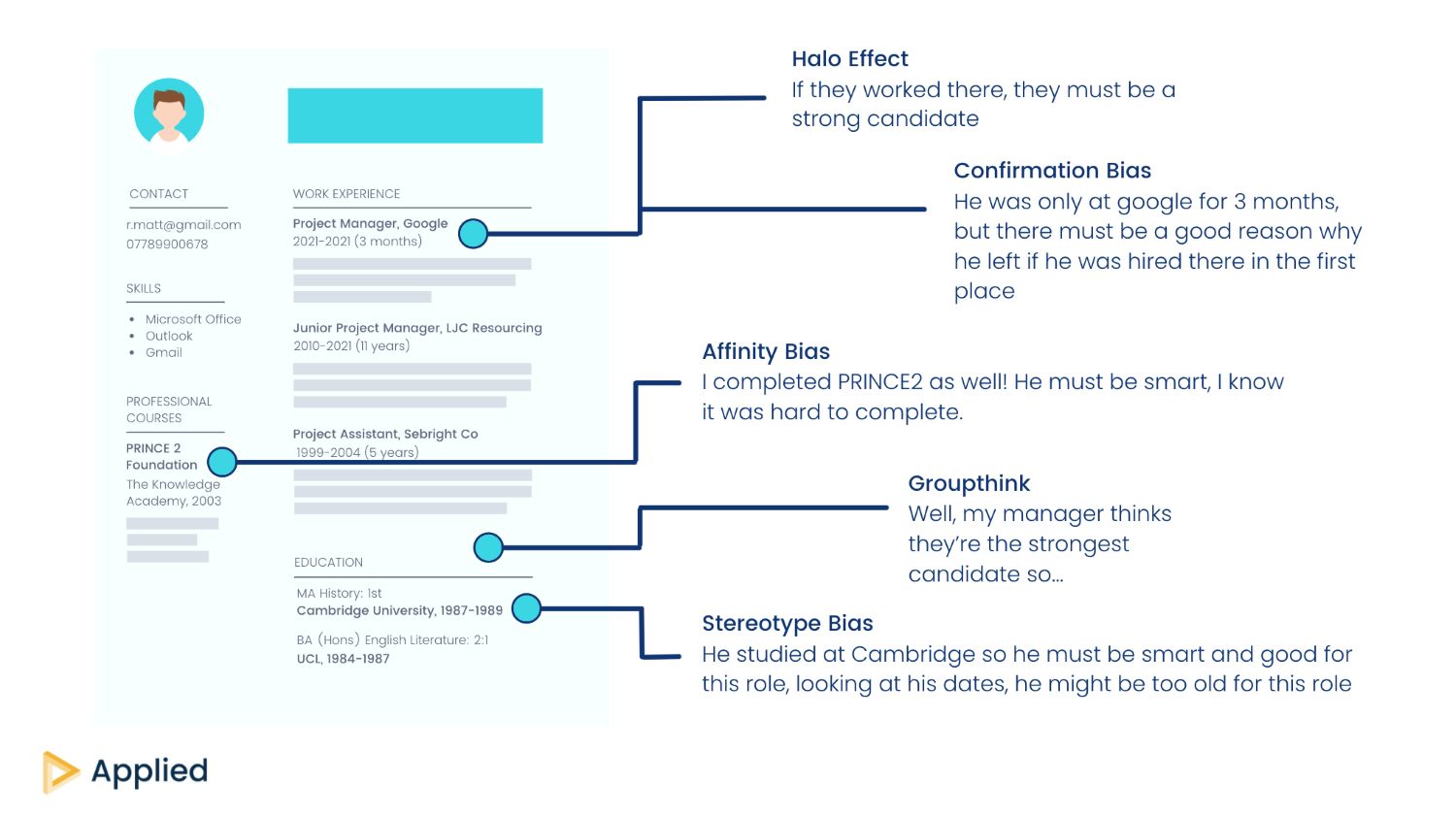
A candidate’s name, address, date of birth can all trigger biases that lead to them being unfairly favoured or overlooked.
So, first things first, anonymous applications are a must.
Although far from ideal (and we'll share our more predictive alternative below), sometimes CVs are necessary if you're hiring for a role beyond your expertise or a very senior position.
If you feel your process still requires CVs, we'd recommend trying structured CV reviews.
Give yourself a list of questions to score candidates against - has this person been promoted in previous roles? Do they have experience in a similar environment?
We built our CV Scoring Tool to make your CV screening as data-driven and ethical as possible. Score candidates using our library of focus questions, with all the data you need to back up your shortlisting decisions.

You’ll notice that on the imagine above, the candidate’s education and experience have been flagged as grounds for bias.
Using skill-based assessments to identify talent
If you want to make your hiring process as bias-proofed as possible, you'll need to look beyond CVs.
CVs might've been around since the 1950s but behavioural science has come a long way since then.
We now have more ethical and predictive assessments at our disposal.
The truth is: education and experience - the key information on a CV - aren't actually actually predictive of real-life ability.
When scanning a CV, a big-name employer or prestigious university may jump out at you, but when we look at the research into their predictive power, education and experience don’t tell us very much at all.

You can read the metastudy the chart above was based on here.
On the chart above, you’ll notice that the most predictive form of assessments are ‘work sample tests.’
This is what we use to screen candidates here at Applied.
We’ve found that organisations using our process hire up 4x more candidates from minority backgrounds.
And 60% of the people hired would’ve been missed via a traditional CV screening.
SO, WHAT ARE WORK SAMPLES? Work samples take parts of the role and simulate them as closely as possible by asking candidates to either perform a task or explain how they’d go about doing so. They’re similar to your typical ‘situational question’ except they pose scenarios hypothetically - focussing on potential over experience.
Work samples rank so highly in terms of predictivity since they essentially get candidates to think as if they were already in the role.
Education and experience aren’t completely useless, but they’re proxies for skills.
Work samples, on the other hand, test for skills directly.
They’re created by taking the skills required for the role, and then thinking of a scenario or task that would test this skill, should the candidate get the job.
Instead of asking candidates if they’ve done something before, work samples simply ask them what they would do.
Here’s an example we recently used to hire a Sales Development Representative:
It's been a busy week and it's now late on Friday afternoon. You have 4 things you've yet to get to this week, but only time to complete two. Imagine for some reason working late/over the weekend is impossible. Which 2 would you do and why? What would you do regarding the others?
There aren’t right or wrong answers. We're just interested in how you think about priorities.
1. There is a Webinar next week, we typically go for 200 sign-ups but it only has 100 so far. Perhaps an extra campaign?
2. Your line manager has asked for a report on how the most recent campaigns have been going as he is chatting with the CEO later that evening.
3. The Community Lead is keen to launch a campaign for next months Training Days first thing on Monday. You haven't written the emails, or got the list of new targets yet.
4. After the last training days you haven't followed up with the attendees to see if they would like to demo the platform. There is about 80 to contact.
Skills tested: Communication, Prioritisation
Instead of a CV/cover letter, we have candidates submit 3-5 of these work samples anonymously.
Once submitted, we then put the answers through a mini-process to ensure things like ordering effects and groupthink are removed from decision-making.
Chunking: applications are sliced up so that you’re comparing candidates’ answers question by question, as opposed to reviewing answers candidate by candidate (one good/bad answer can muddy our perception of the others).
Randomisation: for each question, the order in which answers are reviewed is randomised to avoid ordering biases (applications viewed first tend to be scored higher).
Review: We have three team members score answers against set criteria. This uses the power of crowd wisdom - the rule that collective assessment is more accurate than that of a single person. Answers are scored independently, without conferring.

Data-proof your interviewing
When it comes to interviewing, recruitment bias can be hard to avoid.
However, there are steps you can take to make interviewing more fair and objective.
The first change you’ll want to make is switching to structured interviews
WHAT ARE STRUCTURED INTERVIEWS? Structured interviews entail asking all candidates the same question in the same order - this is to make your interviews to be as uniform as possible.
We recommend using work sample-style questions at the interview stage too.
So where you might usually ask candidates about a time when they did something or showed a certain skills, try asking them how they would tackle that thing.
Hiring is ultimately about assessing potential.
You want to see what people can do, not what they have done.
Sometimes past experience does prepare someone for doing that thing in future, but we’d rather test this directly rather assuming that someone is capable just because they’ve done something before.
Interviews are your chance to see how candidates would think and problem-solve if they were on